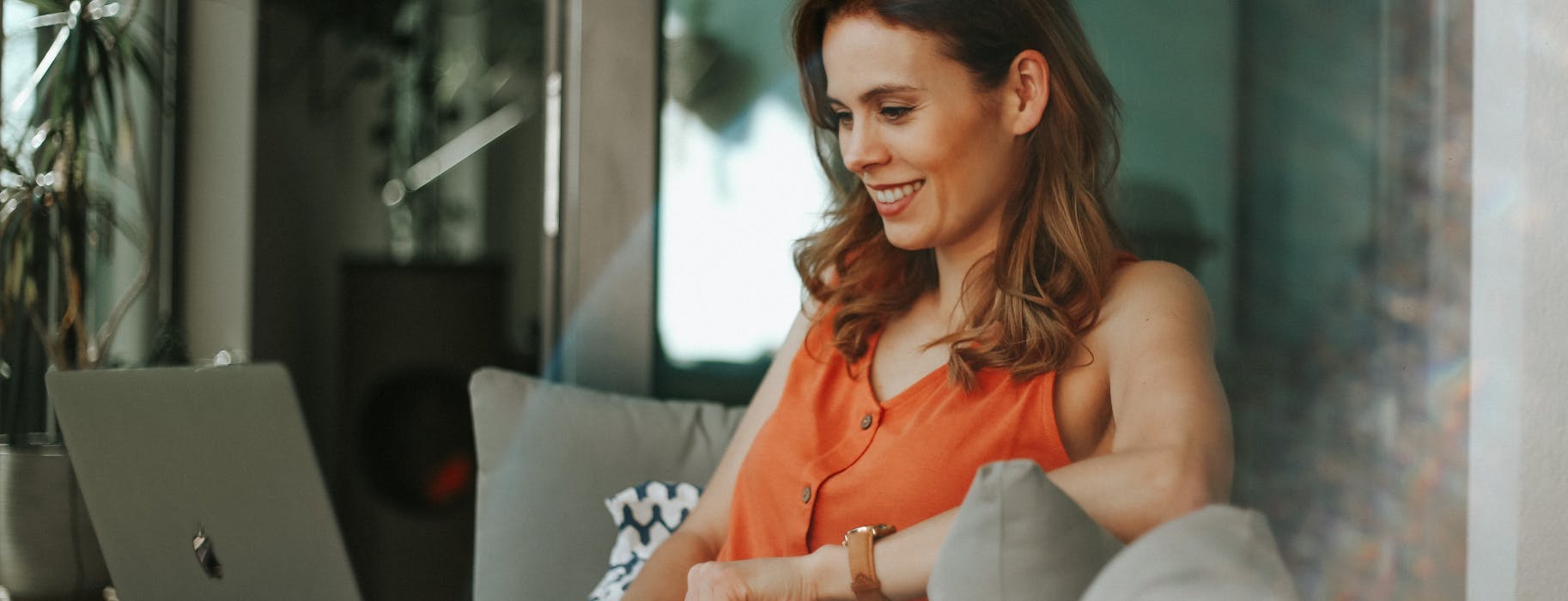
How Can Generative AI Be Used in Cybersecurity? 10 Real-World Examples
In a recent survey of security executives and professionals by Splunk Inc., 91% of respondents said they use generative AI and 46% said it will be game-changing for their security teams.
Despite the relatively recent public availability of generative AI, it is already fundamentally changing the jobs and workflows of cybersecurity professionals.
In this article, we’ll explore the ways generative AI is impacting the cybersecurity industry — for good and bad. We’ll also focus on real-world use cases of generative AI in cybersecurity today.
Recommended reading
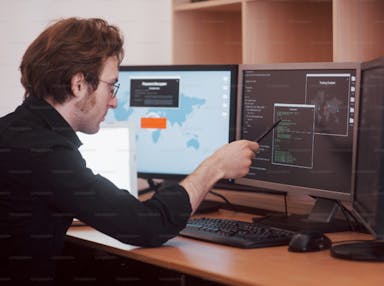
How Artificial Intelligence Will Affect Cybersecurity in 2024 & Beyond
An overview of generative AI in cybersecurity
Generative AI has become a double-edged sword in the realm of cybersecurity. On one hand, malicious actors are increasingly harnessing its power to create sophisticated threats at scale. They exploit AI models like ChatGPT to generate malware, identify vulnerabilities in code, and bypass user access controls. Moreover, social engineers are employing generative AI to craft more convincing phishing scams and deepfakes, amplifying the threat landscape. A substantial 85% of security professionals that witnessed an increase in cyber attacks over the past 12 months attribute the rise to bad actors using generative AI.
However, generative AI also presents significant opportunities for fortifying cybersecurity defenses. It can aid in the identification of potential attack vectors, automatically respond to security incidents, and bolster threat intelligence capabilities.
To fully grasp the impact of generative AI in cybersecurity, CISOs and other security and IT leaders must understand the risks and benefits it offers. We’ll take a closer look at these below.
How generative AI is threatening cybersecurity
Malicious attackers are seizing the potential of generative AI to launch cyber attacks that are harder to detect and defend against. Let’s take a look at some of the risks of generative AI below.
Increasingly sophisticated attacks
In EY’s 2024 Human Risk in Cybersecurity Survey, 85% of respondents said they believe AI has made cybersecurity attacks more sophisticated.
Hackers are using gen AI in particular to launch increasingly sophisticated attacks like self-evolving malware. These strains of malware use gen AI to “self-evolve” and create variations with unique techniques, payloads, and polymorphic code to attack a specific target and go undetected by existing security measures.
Larger volumes of attacks
Hackers are also using gen AI to launch larger volumes of attacks. In a report by Deep Instinct, 75% of security professionals witnessed an increase in attacks over the past 12 months, with 85% attributing this rise to bad actors using generative AI.
That means cybercriminals are using gen aI to create more sophisticated cyber attacks at scale. For example, the IBM X-Force Threat Intelligence Index 2024 found that gen AI capabilities facilitate upwards of a 99.5% reduction in the time needed to craft an effective phishing email.
Lack of risk management
While adoption of gen AI is rising, efforts to manage the risks introduced by gen AI are lagging behind. A recent study released by IBM and Amazon Web Services found that organizations are securing only 24% of their current generative AI projects — despite the fact that 82% of respondents say secure and trustworthy AI is essential to the success of their business. In fact, 69% of the surveyed executives say innovation takes precedence over security.
This is only slightly better than the results of a 2023 report by Riskconnect, in which 93% of companies recognized the risks associated with using generative AI inside the enterprise but only 9% said they’re prepared to manage the threat.
Organizations that increase their adoption of gen AI without simultaneously updating and bolstering their risk management strategy will increase their risk exposure.
Insecure code
Many developers are turning to generative AI to improve their productivity. However, a Stanford study found that software engineers who use code-generating AI systems are more likely to cause security vulnerabilities in the apps they develop. As more developers lacking the expertise or time to spot and remediate code vulnerabilities use generative AI, more code vulnerabilities will be introduced that can be exploited by hackers.
Recommended reading
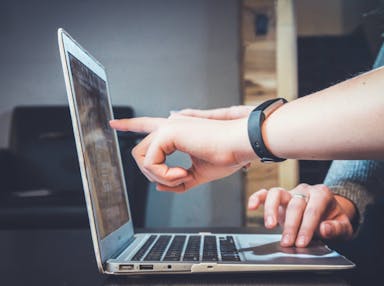
Artificial Intelligence: The Next Big Leap for Security Compliance
How generative AI is improving cybersecurity
Gen AI is also helping make security teams more accurate, efficient, and productive in defending their organizations. Let’s look at how generative AI is transforming security operations below.

Supplementing understaffed security teams
AI is being used to supplement security teams and improve security outcomes. Most IT executives (93%) are already using or considering implementing AI and ML to enhance their security capabilities. These AI adopters are already reporting performance improvements in the triage of Tier 1 threats, detection of zero-day attacks and threats, and reduction of false positives and noise.
As a result of these early indicators of success, more than half of executives (52%) say generative AI will help them better allocate resources, capacity, talent, or skills.
Detecting threats in real time
Threat detection is one of the top use cases of generative AI today. By using it to identify patterns and anomalies faster, more efficiently filter incident alerts, and reject false positives, organizations are able to significantly speed up their ability to detect new threat vectors.
Enhancing threat intelligence
Generative AI is also being used to enhance threat intelligence. Previously, analysts would have to use complex query languages, operations, and reverse engineering to analyze vast amounts of data to understand threats. Now, they can use generative AI algorithms that automatically scan code and network traffic for threats and provide rich insights that help analysts understand the behavior of malicious scripts and other threats.
Automating security patching
Generative AI can automate the analysis and application of patches. Using neural networks, it can scan codebases for vulnerabilities and apply or suggest appropriate patches using natural language processing (NLP) pattern matching or a machine learning algorithm known as the K-nearest neighbors (KNN) algorithm.
Improving incident response
Another successful application of generative AI in cybersecurity is in incident response. Generative AI can provide security analysts with response strategies based on successful tactics used in past incidents, which can help speed up incident response workflows. Gen AI can also continue to learn from incidents to adapt these response strategies over time. Organizations can use generative AI to automate the creation of incident response reports as well.
During the 2024 RSA Conference, Elie Bursztein, Google and DeepMind AI Cybersecurity Technical and Research Lead, said one of the most promising applications of generative AI is speeding up incident response. While more research and innovation needs to be done, he said that one day Gen AI may be able to model an incident or generate a near real-time incident report to help speed up incident response rates drastically.
Recommended reading
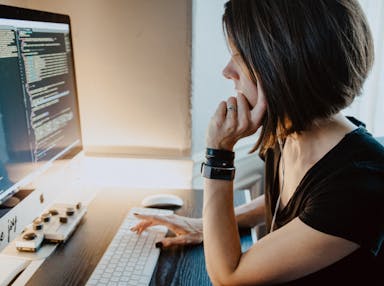
AI in Cybersecurity: How It’s Used + 8 Latest Developments
Generative AI in cybersecurity examples
Now that we understand some of the general applications of generative AI in cybersecurity, let’s take a look at some specific cybersecurity tools that use generative AI.
1. Secureframe Comply AI for Remediation
Secureframe launched Comply AI for Remediation to provide a more contextual, accurate, and tailored user experience for remediating failed tests so organizations can quickly fix cybersecurity issues and speed up time-to-compliance.
Comply AI for Remediation provides remediation guidance that’s tailored to users’ environment and so they can easily update the underlying issue causing the failing configuration in their environment. This enables them to fix failing controls to pass tests, get audit-ready faster, and improve their overall security and compliance posture.
Users can also ask follow-up questions using the chatbot in order to get additional details about the remediation code or to provide more tailored guidance for their specific security and compliance requirements.
2. Google Threat Intelligence
Google recently announced Google Threat Intelligence, which combines the power of Mandiant frontline expertise, VirusTotal threat intelligence which is crowdsourced from over 1 million users, and the Gemini AI model into one offering.
Gemini is an AI-powered agent that provides conversational search across Google’s vast repository of threat intelligence, enabling users to gain insights into threats and protect themselves faster. Traditionally, operationalizing threat intelligence has been labor-intensive and slow. Google Threat Intelligence uses Gemini to analyze potentially malicious code and provides a summary of its findings to more efficiently and effectively assist security professionals in combating malware and other types of threats.
3. Secureframe Comply AI for Risk
Secureframe Comply AI for Risk was designed to automate the risk assessment process to save organizations time and resources.
Using only a risk description and company information, Comply AI for Risk produces detailed insights into a risk, including the likelihood and impact of a risk before a response, a treatment plan to respond to the risk, and the residual likelihood and impact of the risk after treatment. These detailed outputs from Comply AI for Risk help organizations better understand the potential impact of a risk and proper mitigation methods, improving their risk awareness and response.
4. Tenable ExposureAI
Tenable launched ExposureAI to provide new and rich insights to analysts to make exposure management more accessible. These new generative AI capabilities help analysts search, analyze, and make decisions about exposures faster by:
- allowing analysts to use natural language search queries to search for specific exposure and asset data
- summarizing the complete attack path in a written narrative so analysts can understand exposures better
- allowing analysts to ask Tenable’s AI assistant specific questions about the summarized attack path, as well as each node along the attack path
- surfacing high-risk exposure insights and recommending actions so analysts can more easily prioritize and remediate high-risk exposures
5. Ironscales Phishing Simulation Testing
Ironscales launched GPT-powered Phishing Simulation Testing (PST) as a beta feature. This tool uses Ironscales' proprietary large language model to generate phishing simulation testing campaigns that are personalized to employees and the advanced phishing attacks they may encounter.
The goal is to help organizations rapidly personalize their security awareness training to combat the rise and sophistication of social engineering attacks.
6. ZeroFox FoxGPT
ZeroFox has developed FoxGPT, a generative AI tool designed to accelerate the analysis and summarization of intelligence across large datasets. It can help security teams analyze and contextualize malicious content, phishing attacks, and potential account takeovers.
7. SentinelOne Purple AI
SentinelOne unveiled a generative AI-powered threat hunting platform that combines real-time, embedded neural networks and a large language model (LLM)-based natural language interface to help analysts identify, analyze, and mitigate threats faster.
Using natural language, analysts can ask complex threat and adversary-hunting questions and run operational commands to manage their enterprise environment and get rapid, accurate, and detailed responses back in seconds. Purple AI can also analyze threats and provide insights on the identified behavior alongside recommended next steps.
8. VirusTotal Code Insight
VirusTotal Code Insight uses Sec-PaLM, one of the generative AI models hosted on Google Cloud AI, to produce natural language summaries of code snippets. This can help security teams analyze and understand the behavior of potentially malicious scripts. VirusTotal Code Insight is meant to serve as a powerful assistant to cybersecurity analysts, working 24/7 to enhance their overall performance and effectiveness.
9. IBM QRadar Suite
The QRadar suite combines advanced AI and automation to accelerate threat detection and response time. IBM has announced it will release generative AI security capabilities in early 2024 to further automate manual tasks and optimize security teams’ time and talent. These tasks include:
- Creating simple summaries of security cases and incidents that can be shared with a variety of stakeholders in a single click
- Automatically generating searches to detect threats based on natural language descriptions of attack behaviour and patterns
- Helping analysts to quickly understand security log data by providing simple explanations of events that have taken place on a system
- Interpreting and summarizing highly relevant threat intelligence
10. Secureframe Questionnaire Automation
Responding to security questionnaires can be a tedious and time-consuming process for security analysts and other stakeholders, with questions varying from customer to customer and no standardized format, set, or order of questions.
Secureframe’s Questionnaire Automation uses generative AI to streamline and automate the process. This tool suggests questionnaire responses using policies, controls, tests, and other context from the Secureframe platform along with approved prior responses in the Knowledge Base to deliver greater accuracy. After quickly reviewing the answers and making any adjustments as needed, users can then share completed questionnaires back to prospects and customers in their original format.
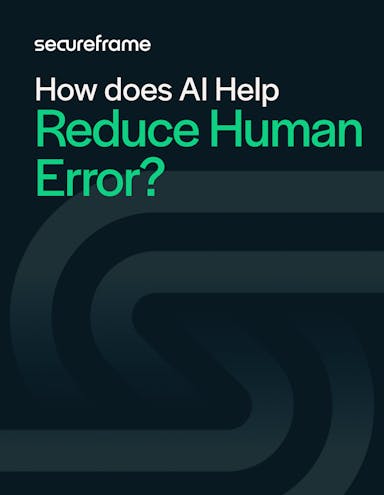
How Does AI Reduce Human Error in Cybersecurity?
This ebook provides a high-level overview of the crucial role AI is playing in mitigating risks of data breaches, regulatory violations, financial losses, and more, using examples of real-world applications in cybersecurity and IT compliance.
What your organization can do in response to generative AI in cybersecurity
Below are some steps your organization can take right now to start defending your organization against generative AI risks.
1. Update employee training
Take the time to evaluate and update employee training around generative AI. This training should reflect the sophistication of cyber attacks leveraging generative AI, including increasingly convincing phishing emails and deep fake calls and videos.
Consider including guardrails for using generative AI tools in employee training as well.
2. Define acceptable generative AI use in policies
While a recent report by ExtraHop revealed that 32% of organizations have banned the use of generative AI tools, leading organizations like the AICPA are recommending that organizations update their security policies to promote safe usage of AI tools since it’s inevitable. Key considerations include:
- providing examples of acceptable use
- limiting usage to one or two reputable tools which the employee has been properly trained upon
- prohibiting the use of any AI chatbot tool that has not been previously vetted by the IT department and approved by the employee’s supervisor
3. Reduce shadow AI
Similar to how shadow IT increased as SaaS products became more popular and accessible, shadow AI is growing as employees are increasingly adopting AI to improve their productivity.
Shadow AI presents major challenges in terms of security and governance for two major reasons. One, employees may expose sensitive, privileged, or proprietary information when using AI products. Two, an organization’s security team can’t assess and mitigate the risks of AI tools that they don’t know about.
To address these challenges, organizations can take a multi-pronged approach to reduce shadow IT. This may include educating employees on the risks of shadow IT, identifying unsanctioned AI services and other guardrails in policies, and implementing offensive and defensive security strategies like security fencing to detect and control what type and how much data is flowing within your organization.
4. Use generative AI to bolster your defenses
Since generative AI is a double-edged sword, make sure to use it to your advantage. For example, you can deploy generative AI solutions to detect threats with the speed, scale, and sophistication as nefarious actors are launching them. You can also use it to automate routine tasks that don’t require as much human expertise or judgment, like threat hunting.
When used strategically in these ways, generative AI and automation can help your organization identify and respond to security risks and incidents faster and at scale.
5. Comply with regulations and frameworks for responsible AI use
AI regulation has already been passed by the EU, China, and parts of the US, and is expected to increase. There are also an increasing number of voluntary frameworks that address the complexities and ethical concerns of AI and are designed to help organizations mitigate risks and enhance governance, like the NIST AI Risk Management Framework (AI RMF) and ISO 42001.
Complying with these regulations and frameworks can provide organizations with a structured approach to managing AI systems responsibly and effectively, thereby enhancing trust and reliability among AI developers and users.
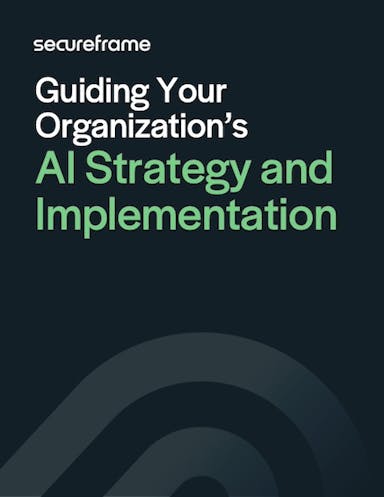
Guiding Your Organization's AI Strategy and Implementation
Follow these best practices to effectively implement AI while addressing concerns related to transparency, privacy, and security.
How Secureframe’s generative AI capabilities can improve cybersecurity at your organization
Secureframe continues to expand its AI capabilities to help customers:
- Respond to security questionnaires and RFPs quickly and accurately to close more deals and grow their revenue
- Automate the risk assessment process to improve their risk awareness and response
- Fix failing controls to pass tests and get audit-ready faster
Want to explore Secureframe AI further? Schedule a demo to see how Secureframe AI can automate manual tasks related to security, privacy, and compliance.
FAQs
How is generative AI used in cybersecurity?
Generative AI is being used to strengthen organizations’ security posture by augmenting security teams’ capabilities to detect, analyze, and respond to threats faster and more efficiently and by automating routine tasks like incident response reporting. Gen AI is also being used by cyber criminals to leverage more and increasingly sophisticated cyber attacks, like deepfake video calls.
What is generative AI and an example?
Generative AI is a type of AI that uses deep-learning models or algorithms to automatically create text, photos, videos, code, and other output based on the datasets they are trained on. The most-well known example is ChatGPT, an AI-powered language model developed by OpenAI.
Can cybersecurity be automated by generative AI?
Parts of cybersecurity can be automated by generative AI, including threat detection, analysis, and response; however, it can’t entirely replace human experts. For example, while generative AI tools can identify known attack patterns and predict new ones, human analysts can confirm real threats from false positives based on their deeper, contextual understanding of their organization’s unique systems, networks, and operational environment.