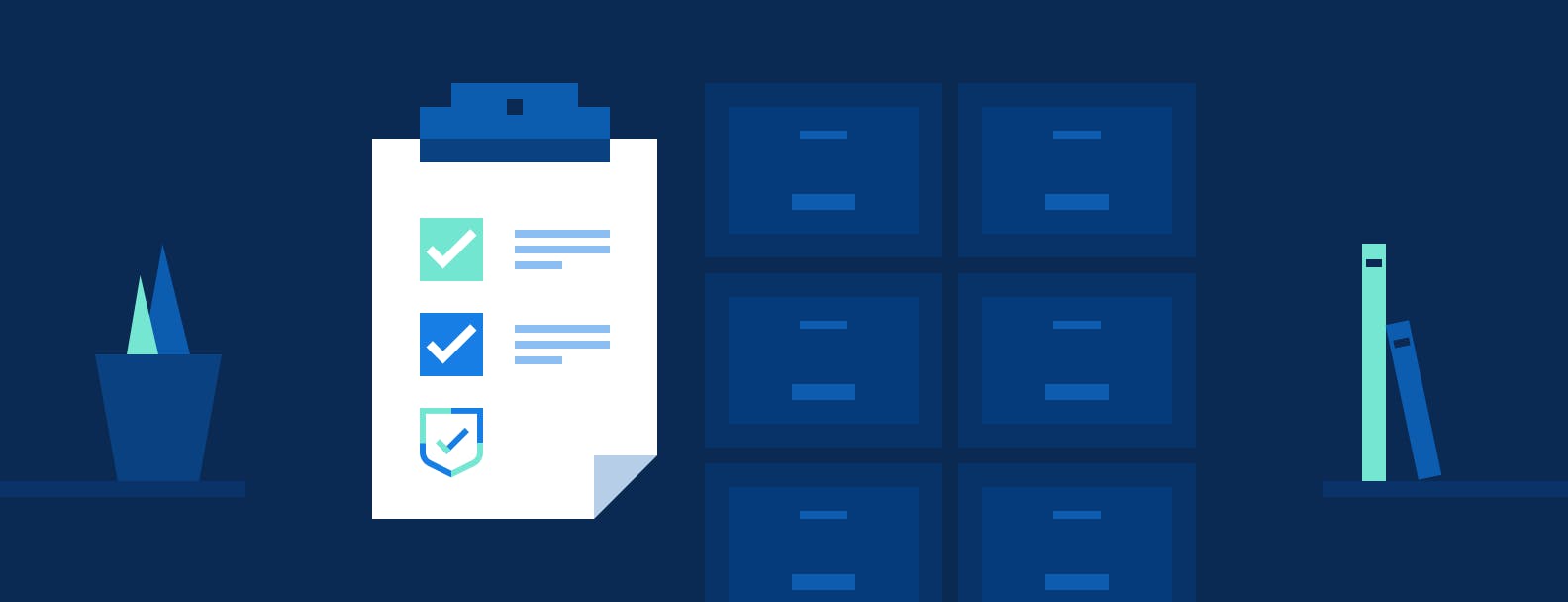
Data Classification: Policy Examples + Template
According to Statista, global data creation is projected to explode over the next decade, growing to more than 180 zettabytes by 2025 and over two thousand zettabytes by 2035.
While only a small percentage of this newly created data is kept, organizations are still facing the challenge of managing more data than ever before and safeguarding it against breaches.
Data classification can help your organization address this challenge, enabling you to sort information according to risk level and set proper data security policies.
Read on to learn how to determine data classification levels, what methods you can use to classify data, what steps and best practices you need to follow to create a data classification policy, and more.
What is data classification?
Data classification is the process of identifying and categorizing data according to its sensitivity level to help simplify the management, security, and storage of that data.
Data classification comes after the data discovery process. So first, you scan your environment to determine where structured and unstructured data resides. It will likely be spread across databases, cloud storage services, and files like PDFs and emails, among other sources. Then, within these discovered data sources, you identify different types of data and assign them labels based on characteristics like:
- Sensitivity: Examples include high, medium, and low-sensitivity.
- Type of data asset: Examples include vendor invoices, customer invoices, and employee records.
- Source information: Examples include licensed, acquired, or internally created.
- Geopolitical information: Examples include US person or EU entities.
These labels are part of an organization’s data classification scheme. Now let’s take a closer look at why data classification is important.
What is the purpose of data classification?
The data your business creates includes everything from valuable intellectual property to calendar invites between coworkers and can pose a real challenge from a risk and data governance perspective. Your business needs a system for organizing both sensitive and low-priority data — that’s where data classification comes into play.
Data classification involves sorting information according to sensitivity level so you can set proper data security policies to mitigate the risk of that being altered, stolen, or destroyed.
Let’s take a closer look at the benefits of data classification so you understand why your organization should start classifying its data.
Benefits of data classification
Effective data classification is a key part of any information security policy and should help your organization safeguard high sensitivity data, streamline data searches and retrieval, remove duplicate data, and comply with data privacy and security requirements
Let’s take a closer look at each of these benefits below.
Risk management
Data classification policies should help you develop a sensible risk management strategy. Once you identify the value of your data, you can implement security measures to minimize the risk of that data being altered, stolen, or destroyed.
Data classification is therefore a key part of risk management and data loss prevention strategies.
Data security and retrieval
Data classification can also be useful for creating data security and retrieval processes by helping you to:
- Organize data by importance
- Safeguard high sensitivity data
- Streamline data searches and retrieval
Doing so can help your organization reduce user access to sensitive data, install the right data protection technologies, and optimize resource utilization for less critical data.
Data deduplication
Data classification can not only help make data more searchable and trackable — it can also help eliminate duplicate data. This can help your employees speed up their search process and also reduce storage and backup costs for your organization as a whole.
Organizational efficiency
Data classification policies can also help improve your organizational efficiency. For example, you can find and cut duplicate data to reduce storage and backup costs.
Regulatory compliance
Data classification can also help your organization comply with data privacy requirements and other rules and regulations by putting appropriate security controls in place and making data searchable and retrievable within specified timeframes.
Now that you know why data classification is worth the effort, we’ll walk you through how it’s accomplished.
Recommended reading
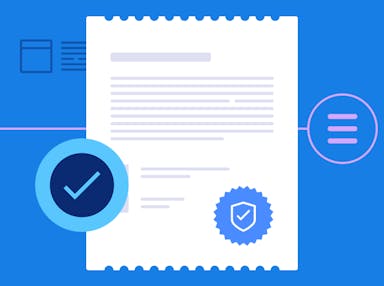
Regulatory Compliance: Benefits and Best Practices to Keep Your Business Safe [+ Checklist]
Data sensitivity classification
Data classification requires you to assess the level of sensitivity of data across your organization. These levels typically range from high to medium to low and correlate to how damaging it would be if that data was lost, stolen, or compromised.
Classifying data in this way helps organizations understand where to focus their risk mitigation efforts. The more sensitive the data is, the more your organization needs to focus on protecting it.
Low sensitivity data
Low sensitivity data is data that would have little to no impact if compromised, lost, or destroyed (although an organization may still put security controls in place to protect against damages). Low sensitivity data is for public use and doesn’t require any confidentiality protections. It is commonly labeled unrestricted or public data, depending on their classification model.
Examples of low sensitivity data include:
- Public information and web pages, such as job postings, blog posts, etc.
- Press releases
- Employee directory
Medium sensitivity data
Medium sensitivity data is data that would not have a catastrophic impact if compromised, lost, or destroyed but would result in some risk to an organization. This data should therefore only be accessible to internal personnel who were granted access and is commonly labeled internal or private.
Examples of medium sensitivity data include:
- Internal emails or documents that don’t contain confidential data
- Supplier contracts
- IT service management or telecommunication information
High sensitivity data
High sensitivity data is data that if compromised, lost, or destroyed would have a catastrophic impact on an organization. Organizations must therefore place the strictest access controls on high sensitivity data. Because access is limited on a need-to-know basis, high sensitivity data is commonly labeled confidential or restricted data.
Examples of high sensitivity data include:
- Financial records, such as credit card numbers
- Medical and biometric data, including protected health information (PHI)
- Employee records, including personally identifiable information (PII) like Social Security numbers
- Authentication data, such as login credentials
Data classification models and schemes
A data classification model and scheme defines how an organization identifies and categorizes its data assets. Typically, these define three to five tiers based on the criticality and sensitivity of data in order to help determine appropriate security controls.
Organizations should design their own data classification models and schemes based on their need to protect proprietary, business, and/or user data with varying levels of sensitivity and to meet compliance and regulatory requirements. However, they can start with or base theirs off different classification models and schemes developed by governments and commercial organizations.
For example, the U.S. government uses a three-tier classification scheme for data based on the potential impact to national security if it is disclosed:
- Confidential: Unauthorized disclosure of this information would likely cause damage to national security.
- Secret: Unauthorized disclosure of this information would likely cause serious damage to national security.
- Top Secret: Unauthorized disclosure of this information would likely cause exceptionally grave damage to national security.
NIST developed a three-tiered categorization scheme based on the potential impact to not just confidentiality but also the integrity and availability of information and information systems applicable to an organization’s mission:
- Low: Unauthorized disclosure of this information would have a limited adverse effect on organization operations, organization assets, or individuals.
- Moderate: Unauthorized disclosure of this information would have a serious adverse effect on organization operations, organization assets, or individuals.
- High: Unauthorized disclosure of this information would have a severe or catastrophic adverse effect on organization operations, organization assets, or individuals.
Organizations can use secondary labels within these tiers to specify different data assets and handling procedures or compliance and regulatory requirements. For example, an organization that only collects financial records may classify that as “confidential data” but an organization that collects medical records may classify that more specifically as “protected health information” in order to indicate that HIPAA requirements apply to that data.
Data classification examples
While the NIST data classification scheme is widely recognized as an adequate classification scheme in sector-specific, national, and international certifications, organizations should develop their own classification schemes based on their unique organizational and risk management needs.
For inspiration, we’ll look at some examples of organizations and the classification model and scheme they have implemented.
UW-Madison
UW-Madison classifies data into four categories, which are used to determine how to provision access to data to individuals. The categories are:
- Public: The unauthorized disclosure, alteration or destruction of this data would result in little or no risk to the University and its affiliates. Any data displayed on websites or published without access restrictions should be classified as public.
- Internal: The unauthorized disclosure, alteration or destruction of this data could result in some risk to the University and its affiliates. By default, any data that is not explicitly classified in the other three categories should be classified as internal.
- Sensitive: The unauthorized disclosure, alteration, loss or destruction of this data could cause a moderate level of risk to the University, affiliates or research projects.
- Restricted: The unauthorized disclosure, alteration, loss or destruction of that data could cause a significant level of risk to the University, affiliates or research projects. If protection of the data is required by law or regulation or UW-Madison is required to self-report to the government and/or provide notice to the individual if the data is inappropriately accessed, then it should be classified as restricted.
Harvard
Harvard classifies data into five levels:
- L1: L1 refers to public information. The University intentionally provides this information to the public. Published research, course catalogs, regulatory and legal findings, published annual reports, released patents, and university-wide policies are all examples.
- L2: L2 refers to low risk confidential information. The University chooses to keep this information private within the Harvard community, but its disclosure beyond the community would not cause material harm. Department policies and procedures, Harvard training materials, drafts of research papers, and patent and grant applications are all examples.
- L3: L3 refers to medium risk confidential information. The University intends to share this information only for those with a “business need to know” and disclosure beyond the intended recipients might cause material harm to individuals or the University. Non-directory student information, non-published faculty and staff information, budget /financial transactions information, and information specified as confidential by vendor contracts and NDAs are all examples.
- L4: L4 refers to high risk confidential information. The University has strict controls for this information and disclosure beyond specified recipients would likely cause serious harm to individuals or the University. Passwords and PINs, system credentials, and private encryption keys are all examples.
- L5: L5 is reserved for research data only, as determined by IRB or Data Use Agreement. Data that, if disclosed, could place the subject at severe risk of harm or data with contractual requirements for exceptional security measures should be classified as L5.
AWS
AWS recommends starting with a three-tiered data classification approach. Both public and commercial organizations that have adopted the AWS cloud have been able to sufficiently meet their data classification needs and requirements using the approach below.
Data classification tier | System security categorization | Cloud deployment model options |
---|---|---|
Unclassified | Low to High | Accredited public cloud |
Official | Moderate to High | Accredited public cloud |
Secret and above | Moderate to Hig | Accredited private/hybrid/community cloud/public cloud |
Data classification methods
There are three primary ways in which your organization can perform data classification. Many organizations use some combination of all three.
Let’s take a brief look at how each method works in practice.
User-based classification
Under user-based classification, you manually decide how to classify files. You can flag sensitive documents when they’re created, after an edit, or before a document is released.
Content-based classification
Content-based classification involves reviewing files and documents for sensitive information before classifying them. A risk category is assigned based on what’s inside each file or document.
Context-based classification
Context-based classification uses metadata instead of content to find indicators of sensitive information.
Examples of metadata include:
- The application that created the file (accounting, financial, or healthcare software)
- The user who created the document (e.g., a member of the accounting department)
- The location where a file was created (e.g., accounting department building)
Both content and context-based classification are types of automated classification. While automated classification tends to be more efficient than user-based classification, you should still verify the results manually. That’s why organizations typically employ two or three of these methods.
Once you determine which classification system is right for your organization, you can kick off your data classification process.
Data classification process
There are some key steps your organization should take during the data classification process.
1. Conduct a risk assessment
To start, you need to think strategically about your data. Where are you vulnerable? How can you optimize your protection?
Here are a few questions that can help you understand your data and what corporate, regulatory, and contractual privacy and confidentiality requirements apply to your organization:
- Who creates or owns the information?
- Who is responsible for the integrity and accuracy of the data?
- Where is the information stored?
- What sensitive data do we have?
- Who has permission to access, change, archive, or delete the information?
- How will it affect our business if the data is stolen, destroyed, or altered?
- Is the information subject to any regulations or compliance/industry standards? If yes, what are the penalties for non-compliance?
After following these practices, you should understand your business’s data better. This will help you develop the best strategy for its management and protection.
2. Define your objectives and what you would like data categorization to achieve.
Next, clearly define your primary goals for data categorization. Do you want to inform regulatory compliance processes, increase employee productivity, or reduce data management and storage costs? All of the above? This step should involve stakeholders from security, compliance, and legal.
3. Determine the categories and criteria you will use to classify data.
Once you understand why you’re classifying your data, you can better determine how to do so. There are multiple ways you can organize data: using metadata, tags, file type, character units, and size of data packets are just a few examples.
You should also establish classification levels at this stage.
4. Formalize a data classification policy.
A data classification policy should clearly outline your organization’s objectives in putting a data classification process in place, the taxonomy that will be used to classify data, and the roles and responsibilities of data owners, including how they classify data and grant access to it.
A data classification policy should clearly outline your organization’s data classification scheme and the formal description of all data types within an organization. The purpose is to enable any affected parties, including external parties who share or receive data, to have a common understanding and identify different types of data.
5. Outline employees’ roles and responsibilities in following data classification protocols.
Employees should clearly understand they’re responsible and accountable for their use of sensitive and low-priority data. Risk mitigation steps and automated policies should be documented. This will allow employees to know to move or archive PHI if unused for 180 days, for example, or how to detect and report control failures or violations.
6. Develop security standards that align with data categories, tags, and compliance regulations.
Once data has been classified by category, tag, and/or compliance regulations, you can determine appropriate security controls for protecting it. For example, medical, credit card, and personally identifiable information (PII) must be handled appropriately for different regulations and therefore may require unique security standards.
7. Periodically re-evaluate your classification criteria and process.
Data classification is not a one and done process. You should periodically review your classification criteria and process as a whole to keep up with changing regulations and business objectives. This may be done on an annual basis or at whatever frequency is possible based on available resources.
Data classification policy examples
To aid in the development of your organization’s data classification policy, check out examples of data classification policies implemented at universities below.
1. The University of Kansas's Data Classification And Handling Policy
This policy outlines how data at the University of Kansas is classified and handled to ensure its confidentiality. It outlines its purpose, who it applies to, and defines three data classification levels based on sensitivity (confidential, sensitive, and public).
2. Boston University's Data Classification Policy
Boston University's Data Classification Policy provides a common vocabulary that individuals can use to describe University data and quantify the amount of protection required. This policy defines four categories into which all University Data can be divided:
- Public
- Internal
- Confidential
- Restricted Use
3. Fordham University’s Data Classification and Protection Policy
Fordham University’s Data Classification and Protection Policy establishes a framework for classifying institutional data based on its level of sensitivity, value, and criticality to the University. It defines three categories: Fordham Protected Data, Fordham Sensitive Data, or Public Data.
Still unsure of what to include in your data classification policy? Use our template as a foundation to quickly create your own.
Data classification best practices
Use these best practices to build an effective data classification policy:
- Understand Your Data: You need to know what kind of data you have. Analyze your data and all regulations that your organization must follow. We’ll take a closer look at these regulations in the next section.
- Create a Data Classification Model: Next, you should build a data classification model. Start with a few basic classification levels. You can add more complex levels as needed.
- Organize Your Data: Decide how to tag your data based on its level of sensitivity and potential impact. As the sensitivity increases from low to high, the classification level should also increase. Add more restrictions at each level.
- Validate your results: All results, whether classified manually or automatically, should be reviewed and validated for accuracy. Create a process that clearly identifies who is involved and what steps are required to review and validate these results.
- Figure out how your results can benefit your organization: Once you’ve validated your results, you can analyze them to determine their best use. Maybe they can be used to streamline workflows or enhance a data security policy that benefits your organization.
- Change classification criteria as needed: Your classification criteria may need to be updated due to changes in business or new regulations. So you should establish a process not only for discovering and classifying new data but also for periodically reviewing your criteria.
Compliance frameworks for data classification
Compliance frameworks can be useful for building your data classification policies. There are several regulatory security frameworks that you should keep in mind when classifying data.
SOC 2
Systems and Organization Controls (SOC) 2 evaluates how a company’s security aligns with the Trust Services Criteria. These criteria include security, availability, confidentiality, processing integrity, and privacy.
This framework helps your organization manage customer data and third-party partner risk management.
While valuable, implementing SOC 2 can be complicated. Secureframe can help simplify your SOC 2 compliance.
HIPAA
The Health Insurance Portability and Accountability Act (HIPAA) created standards for protecting patient health information (PHI).
PHI is considered high-risk data. Healthcare organizations must follow strict cybersecurity practices to comply with HIPAA. You need procedures for classifying the data you collect, use, store, or transmit.
You can learn more about streamlining your HIPAA compliance here.
PCI DSS
The Payment Card Industry Data Security Standard (PCI DSS) requires businesses that handle credit card data to protect cardholders’ information.
Unlike government frameworks, private payment companies (MasterCard, Visa, etc.) enforce PCI DSS compliance.
Learn how you can accelerate your PCI DSS compliance with Secureframe.
GDPR
The General Data Protection Regulation (GDPR) protects the data of European Union citizens.
Under GDPR, any organization that handles an EU citizen’s personal data must have a data classification system. Organizations also need a system for tagging data as public, proprietary, or confidential.
You can get and maintain GDPR compliance securely with Secureframe — learn how.
How Secureframe can help simplify data classification
Secureframe can help you quickly set up a data classification policy that meets security and compliance requirements that apply to your organization and keep it up-to-date. Using the Secureframe platform, you can:
- Start with a data classification policy template that’s been approved by former auditors or bring your own existing policy into the platform
- Define data classification levels within this policy
- Easily tailor this policy using Secureframe’s comprehensive policy editor with AI-powered text revisions
- Once finalized, distribute this policy to employees and track acceptance
- Use employee policy implementation and acceptance as evidence of adherence to corporate controls and framework requirements
- Assign a policy owner and use version control to easily track changes and improve visibility
To learn more about how Secureframe can simplify data classification policy management and other aspects of compliance, request a demo.
FAQs
What is meant by data classification?
Data classification is the process of sorting data into different categories. This allows for easier data management, security, and storage.
What is data classification with example?
Data can be classified by sensitivity, from high to medium to low. High sensitivity data is data that if it were compromised, lost, or destroyed, would have a catastrophic impact on the organization. Examples of high sensitivity data include financial records, personally identifiable information (PII) or protected health information (PHI), authentication data, or proprietary data such as intellectual property.
What are the 3 main types of data classification?
Data can be classified based on sensitivity: high (confidential), medium (internal) and low (public).