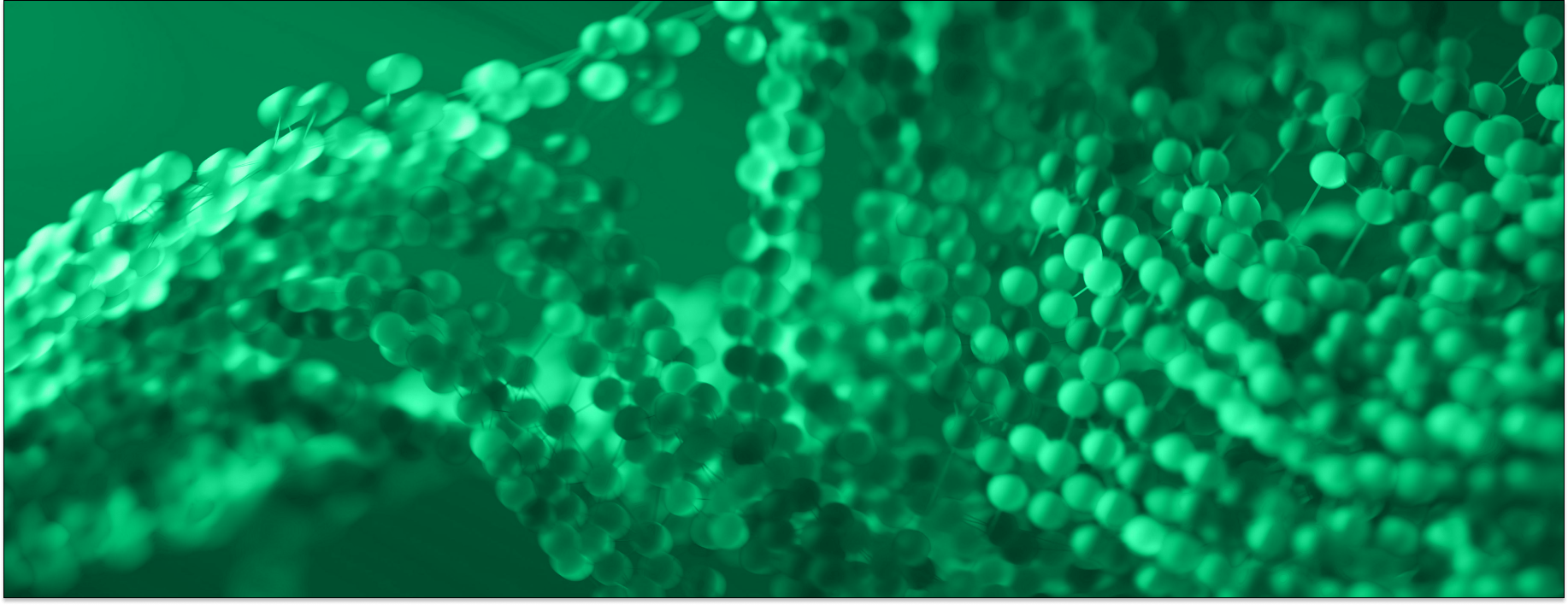
How MSPs and IT Service Providers Can Leverage AI and Machine Learning to Improve Cybersecurity
Emily Bonnie
Senior Content Marketing Manager
Aaron Melear
VP of Partnerships and Alliances
Managed service providers (MSPs) and IT service providers are on the frontlines of the cybersecurity landscape, responsible for protecting their clients’ networks from increasingly sophisticated threats. With the average cost of a data breach reaching $4.88 million in 2024 and cyberattacks increasing by 30% year over year, the pressure on MSPs to maintain airtight security has never been greater.
Yet the sheer volume of incoming alerts MSPs have to manage — an average of 11,000 security alerts per day, according to Forrester — can overwhelm even the most robust IT teams. As attackers employ more advanced tactics like deepfake attacks, adversarial AI, and zero-day exploits, traditional methods of threat detection and mitigation are no longer sufficient.
To address these challenges, MSPs are turning to artificial intelligence and machine learning technologies to enhance their cybersecurity efforts. AI and ML offer a way to scale security operations, streamline compliance management, and automate threat response, allowing IT teams to focus on higher-priority tasks. A recent study found that 69% of organizations believe AI is essential to defending against cyberattacks, making it a critical tool for MSPs aiming to stay ahead of evolving threats.
In this article, we’ll explore how MSPs and IT service providers can leverage AI and ML to improve threat detection, response times, and overall cybersecurity. Find real-world examples of AI-powered tools to learn how AI and ML can help MSPs deliver more efficient, scalable, and adaptive cybersecurity solutions for their clients.
Why AI and ML are game changers for MSPs

AI and ML are not just tools for improving cybersecurity; they represent a transformational shift for MSPs in terms of scalability, operational efficiency, client experience, and profitability. By adopting these technologies, MSPs can move beyond traditional service models and deliver proactive, data-driven, and intelligent solutions that position them as strategic partners in their clients' success.
Scalability and operational efficiency
For MSPs, managing multiple client environments can be resource-intensive, often requiring large teams and a high volume of manual processes to deliver security and IT services. AI and ML enable automation at scale, allowing MSPs to reduce their reliance on manual workflows.
This automation is key for managing repetitive tasks like control monitoring, ticket resolution, or data analysis, freeing up skilled employees to focus on more strategic, high-value tasks. In the long run, AI allows MSPs to service more clients with fewer resources, enhancing profitability without sacrificing quality.
From a business standpoint, this shift means MSPs can improve operational margins while delivering faster, more efficient services. For smaller MSPs looking to scale without proportionally increasing headcount, AI can provide the necessary tools to expand offerings and compete with larger players.
Proactive, predictive service models
Cybersecurity has often involved reacting to threats as they emerge, focusing on mitigating issues after detection. With the introduction of AI and ML, the approach is evolving towards a more predictive and proactive model. These technologies allow for continuous learning and adaptation, enabling MSPs to anticipate potential vulnerabilities and address them before they escalate into major problems.
By leveraging data analytics and machine learning, MSPs can anticipate potential problems or vulnerabilities before they become critical issues, providing preemptive solutions. This shift from reactive to proactive service delivery not only improves customer satisfaction but also positions MSPs as trusted advisors rather than service vendors.
This proactive approach can also reduce downtime and improve business continuity for clients, which enhances the value of MSP services, increasing client trust and loyalty. Predictive capabilities also help MSPs reduce costs related to crisis management and emergency response, as many issues are resolved before they escalate into critical incidents.
Enhanced decision-making and strategic insights
AI and ML offer MSPs access to real-time data analysis and insights that can inform better decision-making, both for the MSP itself and its clients. MSPs can provide actionable intelligence and performance metrics, allowing for more informed decisions about IT investments, risk management, and long-term IT strategies.
For MSPs, this represents an opportunity to transition from service providers to strategic partners. Offering insights and recommendations powered by AI positions MSPs as integral to their clients’ business success, fostering deeper client relationships and potentially opening up new revenue streams, such as consulting or advisory services.
Improved client experience and competitive advantage
As the MSP market becomes increasingly competitive, delivering a superior client experience is essential for differentiation. AI and ML can enable faster response times, more accurate troubleshooting, and a seamless, automated customer journey.
Additionally, MSPs that leverage AI-driven solutions can gain a significant competitive advantage by offering more innovative, adaptive, and comprehensive services. Clients are increasingly seeking providers that can help them navigate digital transformation, and AI-empowered MSPs are well-positioned to deliver cutting-edge solutions.
Cost reduction and profitability
Although the upfront cost of implementing AI and ML technologies can be higher than traditional tools, the long-term financial benefits are substantial. By automating tasks, reducing manual oversight, and improving the accuracy of decision-making processes, MSPs can significantly lower operational costs. For example, AI can help reduce the burden on human resources by handling first-level support or automating compliance reporting.
Ultimately, the efficiencies gained from AI can translate into higher profitability. MSPs can deliver better services at a lower cost, which not only enhances profit margins but also allows them to offer competitive pricing to clients. Over time, this makes AI-powered MSPs more agile and financially resilient in a fast-changing IT landscape.
Adaptability
As client expectations grow and technology evolves, AI and ML give MSPs the tools to adapt more fluidly to future needs. Whether it’s integrating AI into cloud infrastructure management, automating compliance with new regulations, or providing advanced analytics, these technologies future-proof MSP operations. AI enables MSPs to stay at the forefront of technological advancements, ensuring they can meet the complex demands of tomorrow's clients.
Recommended reading
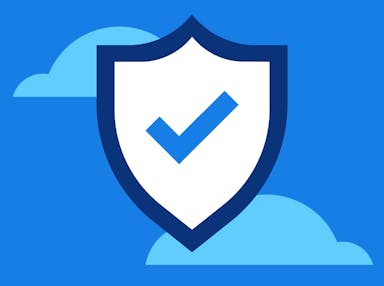
Top 5 Ways to Increase MSP Business Revenue
Real-world AI and ML use cases for MSPs
MSPs and IT service providers manage multiple client environments, each with unique challenges — making scalability and efficiency essential. AI and ML technologies offer a way to not only keep up with the growing volume of compliance tasks, risks and vulnerabilities, and security incidents but also stay ahead of them. These technologies learn from vast amounts of data, identifying patterns and anomalies that would be nearly impossible for human analysts to spot in real-time.
Let’s look at a few practical examples of how MSPs and IT service providers can implement AI and ML to improve their cybersecurity services and client outcomes.
Predictive threat intelligence
Predictive threat intelligence powered by AI and ML enables MSPs to anticipate security threats before they materialize, offering a proactive defense against cyberattacks. AI analyzes historical data and current threat patterns to predict future vulnerabilities and attack vectors. By providing this intelligence in real-time, MSPs can take preventive measures, reducing the likelihood of incidents and minimizing risks for their clients.
For example, AI-driven solutions can use predictive models to forecast and block malware attacks before they occur. An MSP using Cylance was able to prevent a ransomware attack by identifying the malware’s behavior early, well before it could execute on their client’s network. This predictive capability not only protects the MSP’s client from potential damages but also showcases the power of AI in fortifying security postures before issues arise.
Threat detection and anomaly identification
MSPs are required to sift through massive amounts of security data across multiple client environments. AI-powered threat detection offers the ability to process this data at scale, identifying both known and unknown threats faster and more accurately than traditional systems. Unlike signature-based methods that rely on predefined patterns, AI and ML algorithms can detect subtle anomalies in real-time, leveraging both historical data and live inputs. This proactive approach enables MSPs to catch potential threats before they can fully manifest, without the need for constant manual intervention.
For example, machine learning models can learn what "normal" looks like within a network. By continuously learning and evolving its understanding of each environment, it can flag unusual behavior that could signal an attack, such as a device communicating with an unfamiliar IP address or sudden spikes in data transfers.
In one real-world scenario, Darktrace helped an MSP detect a client’s compromised device that was silently communicating with a malicious server overseas. The anomaly was subtle—something that would have likely gone unnoticed by human analysts—but AI flagged it immediately, preventing a potential data breach.
For MSPs managing multiple clients, this capability to scale and adapt in real-time means greater efficiency and accuracy in detecting evolving threats, all while minimizing manual oversight.
Recommended reading
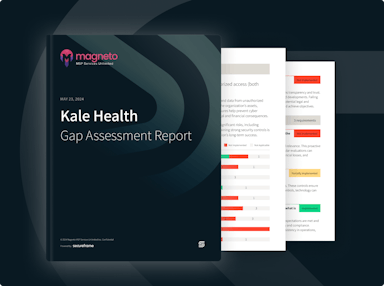
Secureframe Gap Assessment: A Free Tool to Empower Our Service Partners
Automated incident response and mitigation
Responding to cyber threats quickly is critical to minimizing damage, but manual incident response can be slow and resource-intensive. AI enables MSPs to automate many aspects of incident response, allowing for faster neutralization of threats. With predefined response strategies in place, AI can immediately react to common incidents such as malware infections, phishing attacks, or unauthorized access attempts, often before a human analyst is even alerted.
Some solutions integrate AI-driven automation into incident response workflows. For example, if a phishing email is detected on a client's network, the AI can automatically quarantine the affected system, block any further communication from the malicious source, and alert the client’s security team. This reduces the time spent identifying and isolating the threat manually, allowing analysts to focus on more complex or critical threats that require human decision-making.
One MSP used QRadar’s A to stop a ransomware attack in its early stages. When the system detected the ransomware’s unusual encryption activity, it automatically isolated the infected machine from the rest of the network, preventing the malware from spreading further. Without this automated response, the MSP would have had to rely on manual detection and isolation, potentially increasing the scope of the attack and the associated downtime for the client.
By incorporating AI into their incident response strategies, MSPs can reduce response times, limit damage, and free up valuable resources for more in-depth analysis and mitigation.
Enhanced endpoint protection
With the rise of remote work and an increase in the number of connected devices, MSPs must protect endpoints that are scattered across various environments, many of which are no longer within the traditional network perimeter.
AI and ML-based endpoint protection systems provide the ability to continuously learn from device behaviors and recognize deviations that might indicate malicious activity. This dynamic approach is especially important for detecting sophisticated attacks that evade traditional defenses, such as fileless malware or zero-day exploits.
In a SentinelOne case study, a client's IoT device began communicating with an unknown external server, a deviation from its typical usage patterns. SentinelOne’s AI flagged this activity as suspicious, immediately blocked the communication, and alerted the MSP managing the client. Upon further investigation, it was discovered that the device had been compromised and was being used as part of a botnet attack.
This AI-driven approach not only blocked the attack but also provided the MSP with detailed insights into the threat, including the source and behavior of the compromised device. By automating endpoint protection and continuously adapting to new attack methods, AI allows MSPs to protect clients’ distributed and often vulnerable endpoints without constant manual monitoring.
Security and compliance automation
MSPs can use AI-driven security automation to streamline and unify their cybersecurity operations, automating routine tasks such as risk assessments, policy management, vulnerability remediation, and third-party risk monitoring.
For example, AI-enhanced automation tools like Secureframe can automate risk assessments by analyzing risks across multiple client environments, continuously adapting to emerging threats. This not only makes risk assessments faster and more accurate but also ensures MSPs are able to proactively address potential vulnerabilities before they become critical issues. The AI can then generate personalized remediation guidance to quickly patch vulnerabilities across client environments, ensuring that security gaps are closed.
AI also enhances third-party risk management by automating vendor security assessments and compliance monitoring. Given that third-party vendors often represent a significant security risk, having an automated system that continuously evaluates and monitors vendor compliance and security practices is invaluable for reducing supply chain risk. By offering this as part of their services, MSPs can better protect their clients from external threats while demonstrating a proactive approach to risk management.
Recommended reading
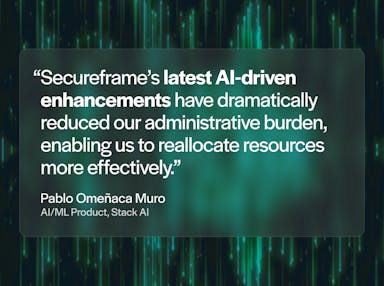
Secureframe Leads AI Innovation in Compliance
Network traffic analysis
AI and ML-driven solutions can monitor network traffic patterns to identify anomalies that might indicate malicious activity. AI can learn the normal behavior of a network and detect unusual traffic that could signify a compromise. For MSPs, this provides an extra layer of defense, especially in monitoring clients’ internal traffic, where breaches might otherwise go unnoticed.
In one instance, Vectra's AI-driven platform helped an MSP discover unusual lateral movement within a client’s network. By detecting these irregular traffic patterns early, the MSP was able to prevent an internal breach from escalating.
Reduced false positives
AI helps MSPs reduce the number of false positives by continuously learning from data. As the system processes more information, it fine-tunes its alert mechanisms to minimize unnecessary alerts, allowing MSPs to focus on real threats.
For example, AI-powered systems can prioritize alerts based on context and severity, which helps streamline the process for security teams and ensures that attention is directed to genuine threats, not noise. This increases efficiency and reduces alert fatigue for security teams, improving overall response times.
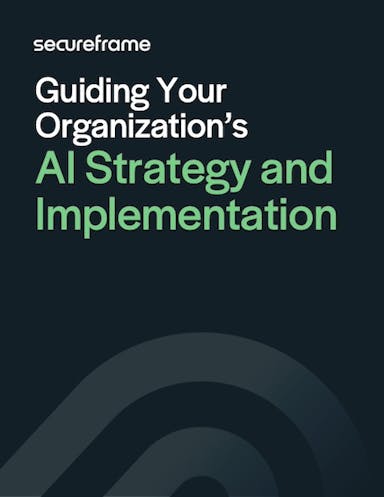
Guiding Your Organization's AI Strategy and Implementation
Follow these best practices to effectively implement AI while addressing concerns related to transparency, privacy, and security.
Overcoming challenges with AI in cybersecurity
While AI and ML bring a new level of sophistication to cybersecurity, they are not without challenges. AI is often overhyped, with some vendors making exaggerated claims. Not every AI solution will live up to its promise, and even the most advanced technologies have limitations.
Data quality and privacy concerns, algorithmic bias, false positives, and the introduction of new security vulnerabilities are all significant challenges for companies looking to incorporate AI into their tech stack. MSPs and IT service providers must be proactive in managing these concerns to ensure AI and ML technologies deliver maximum value.
Here’s how MSPs can address each challenge:
Data quality
AI systems rely heavily on high-quality, comprehensive datasets to function optimally. For MSPs, this means ensuring that their clients' environments provide the volume and variety of data needed to train machine learning algorithms effectively.
By ensuring that datasets are both comprehensive and clean, MSPs can improve the accuracy and performance of AI-powered threat detection systems, leading to better security outcomes for their clients.
MSPs and IT service providers can achieve this by:
- Conducting regular data audits: Periodically audit the quality and completeness of the data flowing through your systems to ensure it aligns with the needs of your AI tools.
- Implementing data normalization: Standardize data formats across different sources to ensure consistency and accuracy in the data fed into AI systems.
- Leveraging diverse data sources: Encourage the integration of data from various endpoints, such as firewalls, IDS/IPS, cloud services, and user behavior analytics, to create a more holistic view of the network. This will enhance AI’s ability to detect anomalies more effectively.
Data privacy concerns
AI systems require huge volumes of data, posing significant data privacy risks. AI models that are used to analyze customer or user behavior, for example, may need access to sensitive personal information. GenAI tools may also share user data with third parties and service providers, potentially violating data privacy laws. Regulation has already been implemented in the EU and China, with proposed regulations in the US, UK, Canada, and India.
MSPs will need to emphasize AI data privacy in their data governance, including data anonymization techniques that preserve user privacy without impacting data utility. Proper AI governance will also help monitor AI performance, detect stale models, and identify bias. As privacy regulations expand globally, MSPs must stay compliant and ensure their AI practices align with legal frameworks.
Potential for bias
When data sets used to train AI and machine learning algorithms are not diverse or comprehensive enough, it can negatively impact the AI model’s performance. Threats can be overlooked, or benign behavior can be identified as malicious.
Imagine an AI-based Intrusion Detection System that’s trained primarily on a dataset of the most common recent cyberattacks, such as malware or ransomware attacks.
This AI system might be highly efficient at detecting similar types of attacks in the future. But as the cyber threat landscape evolves and new attacks emerge, the system may fail to recognize and respond to these threats.
MSPs must continuously update AI models and datasets to ensure they're effective in addressing the ever-changing cybersecurity landscape. Regular audits of AI systems are necessary to minimize bias and maintain reliable threat detection.
False positives
One of the common challenges in AI-based cybersecurity is managing false positives. While AI can reduce the frequency of false alarms compared to traditional methods, there is still a learning curve as the system refines its detection parameters. Left unchecked, false positives can overwhelm security teams and disrupt operations.
To combat this issue, MSPs can:
- Maintain human oversight in early stages: During the initial deployment of AI tools, assign security analysts to manually review AI-generated alerts. This human oversight will help fine-tune detection algorithms, reducing false positives over time.
- Establish continuous feedback loops: Establish a feedback loop between human analysts and the AI system to ensure that the machine learning model learns from mistakes, improving its accuracy with each iteration.
- Prioritize alerts: Utilize AI tools that include prioritization features, which rank alerts based on severity and context. This allows security teams to focus on the most critical threats while filtering out low-risk false alarms.
By taking a hands-on approach during the early stages of AI implementation, MSPs can help the system become more reliable and reduce the risk of alert fatigue.
Upfront costs and integration
AI-based cybersecurity solutions often come with higher upfront costs compared to traditional tools, and integrating these systems into an existing security infrastructure can present challenges. When implemented strategically, the benefits—including reduced manual workloads, faster response times, and improved security outcomes—can quickly outweigh the initial investment.
MSPs can better manage these costs and maximize ROI by:
- Developing an ROI model: Calculate the potential return on investment (ROI) by considering the reduction in manual labor, faster incident response times, and the ability to prevent costly breaches. Present this model to clients to justify the higher initial cost.
- Choosing a phased implementation: Instead of a full-scale deployment, implement AI tools in phases. Start by integrating AI into high-priority areas (e.g., endpoint security or network traffic analysis) and gradually expand as the benefits become clear.
MSPs can overcome the barrier of costs and complexity by creating a roadmap for phased implementation and focusing on clear, measurable benefits that AI brings to security operations.
Recommended reading
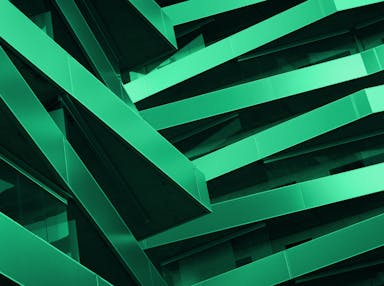
Maximizing Efficiency in GRC Practices for MSSPs
The future of AI in MSP cybersecurity services
AI and ML are still evolving, and their potential in cybersecurity is far from fully realized. Future advancements will likely include more predictive models that anticipate attacks before they occur, even more personalized protection based on specific client environments, and deeper integration with other IT service management tools.
For MSPs and IT service providers, staying ahead of the curve with AI and ML is quickly becoming a necessity to provide clients with fast, efficient, and highly adaptive security services. By embracing AI and ML, MSPs can not only enhance their threat detection and response capabilities but also position themselves as leaders in the increasingly competitive cybersecurity space.
Secureframe is an established leader in AI-powered security automation, with AI and ML workflows that maximize efficiency across risk assessments, cloud remediation, control mapping, vendor management, and more. In addition to supporting key AI security frameworks such as NIST AI RMF and ISO 42001, Secureframe offers:
- Comply AI for Risk simplifies risk assessments by analyzing potential threats and providing actionable insights, including an inherent risk score, suggested treatment plan, and residual risk score.
- Comply AI for Remediation automates the remediation process, providing intelligent recommendations to address compliance gaps. By prioritizing the most critical issues and suggesting effective solutions, it accelerates the path to compliance and reduces the manual burden on security teams.
- Comply AI for Policies helps organizations create, update, and maintain compliance policies automatically. Using AI-driven insights, Comply AI for Policies ensures that documentation stays current with the latest regulations, minimizing the risk of non-compliance and saving time on policy management.
- Comply AI for Control Mapping maps common controls to multiple frameworks, simplifying multi-framework compliance. By reducing duplication and streamlining audits, it helps organizations meet diverse regulatory requirements with minimal effort.
- Comply AI for TPRM helps MSPs and IT service providers assess and monitor third-party risks more effectively. By automating vendor risk assessments and continuously analyzing supplier data, it ensures a more secure supply chain with less manual effort.
- AI Questionnaire Response drastically reduces the time spent completing compliance questionnaires by automating responses. Leveraging existing data and machine learning, it ensures accuracy and consistency across questionnaires, freeing up resources and improving response times.
- AI Vendor Security Reviews quickly analyzes and assesses security controls and compliance documentation from third-party vendors. By identifying potential risks and vulnerabilities in real-time, it streamlines the vendor onboarding process, ensuring that partners meet security standards with less manual oversight.
Discover how Secureframe's AI-powered solutions can elevate your cybersecurity and compliance services. Learn more about our Partner Program to see how we can help you streamline compliance, protect client data, and enhance your security offerings.