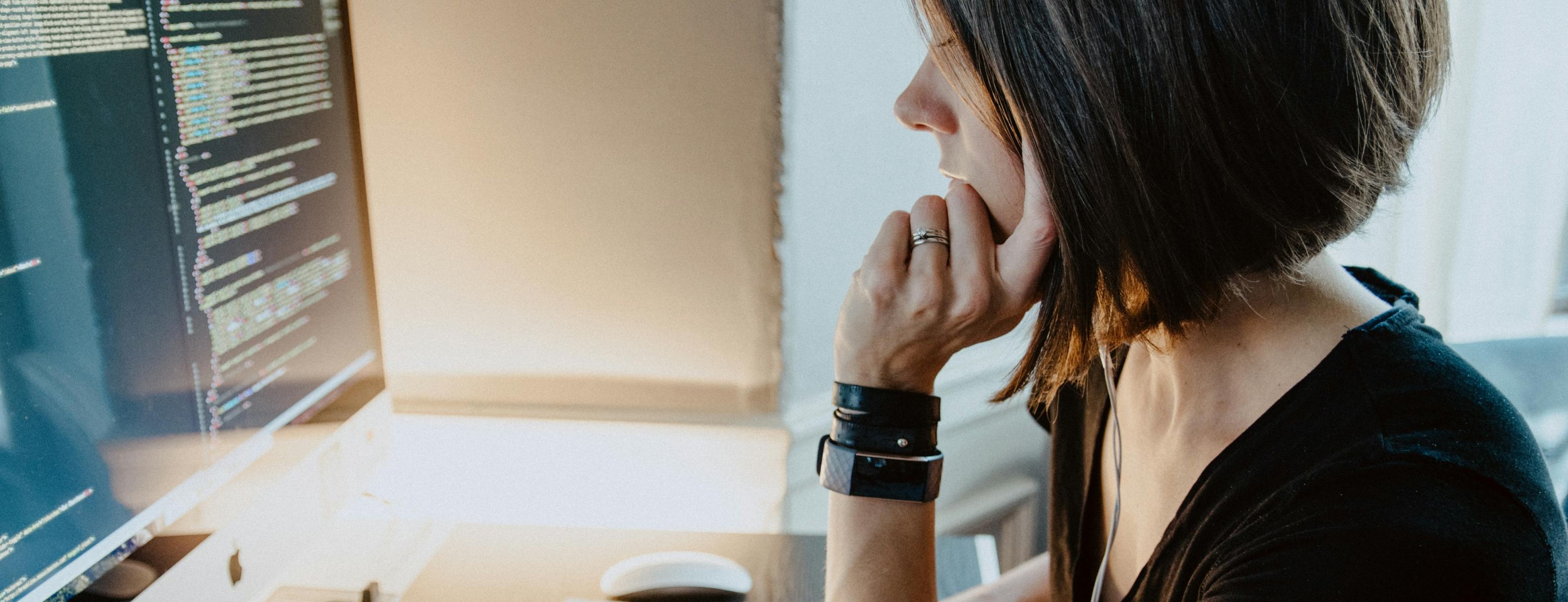
AI in Cybersecurity: How It’s Used + 8 Latest Developments
Artificial intelligence (AI) and machine learning technologies have been powering some cybersecurity capabilities for decades. Anti-virus, spam-filtering, and phishing-detection tools are just a few examples.
However, the recent advances in AI have led to an explosion in interest around AI-powered cybersecurity capabilities. This has resulted in an unprecedented amount of product releases, investment, and discourse around AI in cybersecurity.
To understand how AI has already and will continue to shape cybersecurity, we’ll explain how AI is used in cybersecurity, starting with more established use cases as well as some of the latest developments.
How is AI used in cybersecurity?
AI is used in cybersecurity to automate tasks that are highly repetitive, manually-intensive, and tedious for security analysts and other experts to complete. This frees up time and resources so cybersecurity teams can focus on more complex security tasks like policymaking.

Take endpoint security for example. Endpoint security refers to the measures an organization puts in place to protect devices like desktops, laptops, and mobile devices from malware, phishing attacks, and other threats. To supplement the efforts of human experts and policies they put in place to govern endpoint security, AI can learn the context, environment, and behaviors associated with specific endpoints as well as asset types and network services. It can then limit access to authorized devices based on these insights, and prevent access entirely for unauthorized and unmanaged devices.
Since AI can enhance other areas of cybersecurity as well, there is expected to be an explosion in AI-based cybersecurity products. In 2021, the global market for AI-based cybersecurity products reached $14.9 billion — it is estimated to reach $133.8 billion by 2030.
Before taking a closer look at the use of AI in cybersecurity, let’s take a closer look at the benefits.
Benefits of AI in Cybersecurity
Cybersecurity presents unique challenges, including a constantly evolving threat landscape, vast attack surface, and significant talent shortage.
Since AI can analyze massive volumes of data, identify patterns that humans might miss, and adapt and improve its capabilities over time, it has significant benefits when applied to cybersecurity, including:
- Improving the efficiency of cybersecurity analysts
- Identifying and preventing cyber threats more quickly
- Effectively responding to cyber attacks
- Reducing cybersecurity costs
Consider the impact of security AI and automation on average data breach costs and breach lifecycles alone. According to a survey by IBM, organizations that use security AI and automation extensively report an average cost of a data breach at $3.60 million, which was $1.76 million less than breaches at organizations that didn’t use security AI and automation capabilities. This is a 39.3% difference in average breach cost. Organizations with fully deployed security AI and automation were also able to identify and contain a data breach 108 days faster than companies with no security AI and automation deployed.

Even organizations with limited use of security AI and automation reported an average cost of a data breach of $4.04 million, which was $1.32 million less or a 28.1% difference compared to no use. Organizations with limited use also saw a significant acceleration in the time to identify and contain a breach, with an average of 88 days faster than organizations with no use of security AI and automation.
To better understand the impact of AI on cybersecurity, let’s take a look at some specific examples of how AI is used in cybersecurity below.
Recommended reading
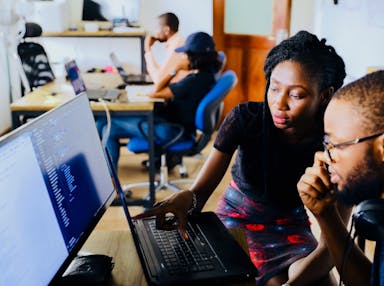
35+ AI Statistics to Better Understand Its Role in Cybersecurity [2023]
AI in cybersecurity examples
Many organizations are already using AI to help make cybersecurity more manageable, more efficient, and more effective. Below are some of the top applications of AI in cybersecurity.
1. Threat detection
Threat detection is one of the most common applications of AI in cybersecurity. AI can collect, integrate, and analyze data from hundreds and even thousands of control points, including system logs, network flows, endpoint data, cloud API calls, and user behaviors. In addition to providing greater visibility into network communications, traffic, and endpoint devices, AI can also recognize patterns and anomalous behavior to identify threats more accurately at scale.
For example, legacy security systems analyzed and detected malware based on signatures only whereas AI- and ML-powered systems can analyze software based on inherent characteristics, like if it’s designed to rapidly encrypt many files at once, and tag it as malware. By identifying anomalous system and user behavior in real time, these AI- and ML-powered systems can block both known and unknown malware from executing, making it a much more effective solution than signature-based technology.
2. Threat management
Another top application of AI in cybersecurity is threat management.
Consider that 59% of organizations receive more than 500 cloud security alerts per day and 38% receive more than 1,000, according to a survey by Orca Security. 43% of IT decision makers at these organizations said more than 40% of alerts are false positives and 49% said more than 40% are low priority. Despite 56% of respondents spending more than 20% of their day reviewing alerts and deciding which ones should be dealt with first, more than half (55%) said their team missed critical alerts in the past due to ineffective alert prioritization.
This results in a range of issues, including missed critical alerts, time wasted chasing false positives, and alert fatigue which contributes to employee turnover.
In order to combat these issues, organizations can use AI and other advanced technologies like machine learning to supplement the efforts of these human experts. AI can scan vast amounts of data to identify potential threats and filter out non-threatening activities to reduce false positives at a scale and speed that human defenders can’t match.
By reducing the time required to analyze, investigate, and prioritize alerts, security teams can spend more time remediating these alerts, which takes three or more days on average according to 46% of respondents in the Orca Security survey.
3. Threat response
AI is also used effectively to automate certain actions to speed up incident response times. For example, AI can be used to automate response processes to certain alerts. Say a known sample of malware shows up on an end user’s device. Then an automated response may be to immediately shut down that device’s network connectivity to prevent the infection from spreading to the rest of the company.
AI-driven automation capabilities can not only isolate threats by device, user, or location, they can also initiate notification and escalation measures. This enables security experts to spend their time investigating and remediating the incident.
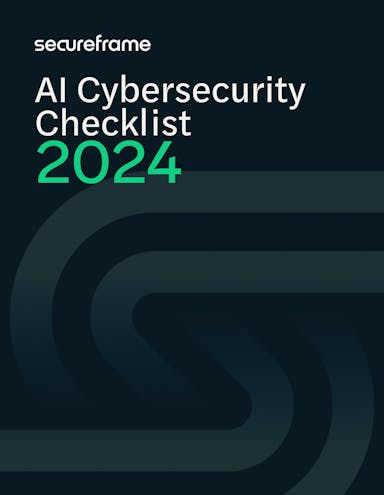
2024 AI Cybersecurity Checklist
Download this checklist for more step-by-step guidance on how you can harness the potential of AI in your cybersecurity program in 2024 and beyond.
Latest developments in cybersecurity AI
When asked what they would like to see more of in security in 2023, the top answer among a group of roughly 300 IT security decision makers was AI. Many cybersecurity companies are already responding by ramping up their AI-powered capabilities.
Let’s take a look at some of the latest innovations below.
1. AI-powered remediation
More advanced applications of AI are helping security teams remediate threats faster and easier. Some AI-powered tools today can process security alerts and offer users step-by-step remediation instructions based on input from the user, resulting in more effective and tailored remediation recommendations.
Secureframe Comply AI does just that for failing cloud tests. Using infrastructure as code (IaC), Comply AI for remediation automatically generates remediation guidance tailored to users’ environment so they can easily update the underlying issue causing the failing configuration in their environment. This enables them to fix failing controls to pass tests, get audit-ready faster, and improve their overall security and compliance posture.
Recommended reading
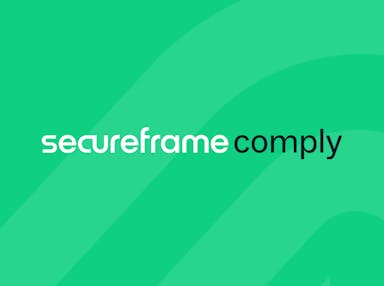
Introducing Secureframe Comply AI: Faster, Tailored Cloud Remediation
2. Enhanced threat intelligence using generative AI
Generative AI is increasingly being deployed in cybersecurity solutions to transform how analysts work. Rather than relying on complex query languages, operations, and reverse engineering to analyze vast amounts of data to understand threats, analysts can rely on generative AI algorithms that automatically scan code and network traffic for threats and provide rich insights.
Google’s Cloud Security AI Workbench is a prominent example. This suite of cybersecurity tools is powered by a specialized AI language model called Sec-PaLM and helps analysts find, summarize, and act on security threats. Take VirusTotal Code Insight, which is powered by Security AI Workbench, for example. Code Insight produces natural language summaries of code snippets in order to help security experts analyze and explain the behavior of malicious scripts. This can enhance their ability to detect and mitigate potential attacks.
Recommended reading
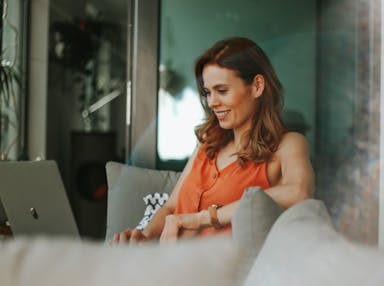
Generative AI in Cybersecurity: How It’s Being Used + 8 Examples
3. Security questionnaire automation via generative AI
Security questionnaires are a common way to vet potential vendors and other third parties to assess whether their cybersecurity practices meet internal and external requirements. While these are important for vendor risk management, they can take up valuable time. AI can help speed up this process by suggesting answers based on previously answered questionnaires. Some more powerful AI tools can even pull from an organization's security policies and controls when suggesting answers to be as accurate as possible.
Secureframe Questionnaire Automation, for example, uses generative AI to suggest answers that draw from an organization's policies, tests, and controls as well as previous responses stored in their Knowledge Base to further enhance accuracy and time savings for users. It’ll even rephrase answers using context hosted in their Knowledge Base. By leveraging AI to automate the collection of answers to security questionnaires from multiple sources and intelligently parse and rephrase responses, this AI solution ensures that answers are consistent, accurate, and tailored to the specific requirements of each question and helps save organizations hundreds of hours answering tedious security questionnaires.
4. Stronger password security using LLMs
According to new research, AI can crack most commonly used passwords instantly. For example, a study by Home Security Heroes proved that 51% of common passwords can be cracked by AI in under a minute.
While scary to think of this power in the hands of hackers, AI also has the potential to improve password security in the right hands. Large language models (LLMs) trained on extensive password breaches like PassGPT have the potential to enhance the complexity of generated passwords as well as password strength estimation algorithms. This can help improve individuals’ password hygiene and the accuracy of current strength estimators.
5. Dynamic deception capabilities via AI
While malicious actors will look to capitalize on AI capabilities to fuel deception techniques such as deepfakes, AI can also be used to power deception techniques that defend organizations against advanced threats.
Deception technology platforms are increasingly implementing AI to deceive attackers with realistic vulnerability projections and effective baits and lures. Acalvio’s AI-powered ShadowPlex platform, for example, is designed to deploy dynamic, intelligent, and highly scalable deceptions across an organization’s network.
6. Simplified vendor questionnaires using AI
Vendor assessments are a crucial aspect of vendor risk management, helping organizations assess the security practices, compliance, and risks of potential vendors before establishing business relationships. Traditionally, these assessments have been manual processes that take up a significant amount of an organization’s time and resources. Since AI is capable of analyzing massive amounts of data much faster than humans can, AI tools can significantly simplify and speed up vendor assessments.
Comply AI for VRM, for example, streamlines the security assessment process. Secureframe customers can not only send custom or template-based questionnaire templates directly from the Secureframe platform and have vendor responses be posted back in the platform automatically for centralized management — they can also use Comply AI for VRM to automatically extract relevant answers from hosted vendor documents, like SOC 2 reports. This saves vendors time from having to respond to every single question, enhancing the efficiency and accuracy of security reviews.
7. AI-assisted development
In 2023, CISA published a set of principles for the development of secure by design products. The goal is to reduce breaches, improve the nation’s cybersecurity, and reduce developers’ ongoing maintenance and patching costs. However, it will likely increase development costs.
As a result, developers are starting to rely on AI-assisted development tools to reduce these costs and improve their productivity while creating more secure software. GitHub Copilot is a relatively new but promising example. In a survey of more than 2,000 developers, developers who used GitHub Copilot completed a task 55% faster than the developers who didn’t.
8. AI-based patch management
As hackers continue to use new techniques and technologies to exploit vulnerabilities, manual approaches to patch management can’t keep up and leave attack surfaces unprotected and vulnerable to data breaches. Research in Action1’s 2023 State of Vulnerability Remediation Report found that 47% of data breaches resulted from unpatched security vulnerabilities, and over half of organizations (56%) remediate security vulnerabilities manually.
AI-based patch management systems can help identify, prioritize, and even address vulnerabilities with much less manual intervention required than legacy systems. This allows security teams to reduce risk without increasing their workload.
For example, GitLab recently released a new security feature that uses AI to explain vulnerabilities to developers — with plans to expand this to automatically resolve them in the future.
9. Automated penetration testing
Penetration testing is a complex, multi-step process that involves gathering information about a company’s environment, identifying threats and vulnerabilities, and then exploiting those vulnerabilities to try to gain access to systems or data. AI can help simplify these parts of the process by quickly and efficiently scanning networks and gathering other data and then determining the best course of action or exploitation pathway for the pen tester.
Although a relatively nascent area of AI cybersecurity, there are already a mix of open-source tools like DeepExploit and proprietary tools like NodeZero offering a faster, more affordable, and scaleable alternative to traditional penetration testing services. DeepExploit, for example, is a fully automated penetration testing tool that uses machine learning to enhance several parts of the pen testing process, including intelligence gathering, threat modeling, vulnerability analysis, and exploitation. However, it is still in beta mode.
10. AI-powered risk assessments
AI is also being used to automate risk assessments, improving accuracy and reliability and saving cybersecurity teams significant time. These types of AI tools can evaluate and analyze risks based on existing data from a risk library and other data sources, and automatically generate risk reports.
Secureframe Comply AI for Risk, for example, can produce detailed insights into a risk with a single click, leveraging only a risk description and company information. This AI-powered solution can determine the likelihood and impact of a risk before a response, suggest a treatment plan to respond to the risk, and define the residual likelihood and impact of the risk after treatment. These detailed outputs from Comply AI for Risk help organizations better understand the potential impact of a risk and proper mitigation methods, improving their risk awareness and response.
Recommended reading
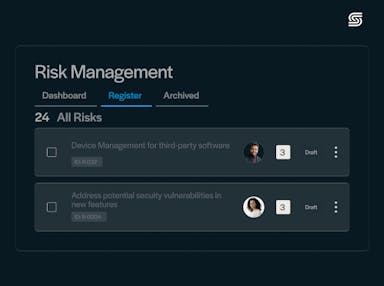
Confidently Grow Your Organization and Reduce Risk with Secureframe’s New Risk Management
AI and cybercrime
While AI is being applied in many ways to improve cybersecurity, it is also being used by cyber criminals to launch increasingly sophisticated attacks at an unprecedented pace.
In fact, 85% of security professionals that witnessed an increase in cyber attacks over the past 12 months attribute the rise to bad actors using generative AI.
As a result of AI-driven cyber attacks as well as other factors, cybercrime is expected to cost $10.5 trillion globally by 2025.
Below are just a few ways that AI is being used in cybercrime:
- Social engineers are using ChatGPT to craft more believable and legitimate sounding phishing emails.
- Social engineers are also using machine-learning algorithms combined with facial-mapping software to create convincing deepfakes.
- Malicious actors are using AI to launch more machine-speed attacks, ie. ransomware and other automated attacks that propagate and/or mutate very quickly and are virtually impossible to neutralize using human-dependent response mechanisms.
- Hackers are using AI-supported password guessing and CAPTCHA cracking to gain unauthorized access to sensitive data.
- Threat actors are creating AI that can autonomously identify vulnerabilities, plan and carry out attack campaigns, use stealth to avoid defenses, and gather and mine data from infected systems and open-source intelligence.
Organizations that extensively use AI and automation to enhance their cybersecurity capabilities will be best positioned to defend against the weaponized use of AI by cybercriminals. In a study by Capgemini Research Institute, 69% of executives say that AI results in higher efficiency for cybersecurity analysts in the organization. 69% also believe AI is necessary to effectively respond to cyberattacks. Find more statistics about the positive impact of AI in cybersecurity.
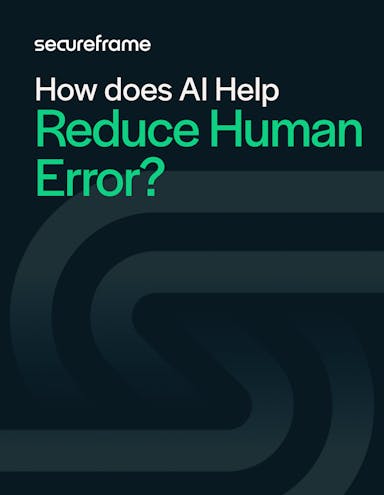
How Does AI Reduce Human Error in Cybersecurity?
This ebook provides a high-level overview of the crucial role AI is playing in mitigating risks of data breaches, regulatory violations, financial losses, and more, using examples of real-world applications in cybersecurity and IT compliance.
How is cybersecurity AI being improved?
In response to these emerging threats, cybersecurity AI is being continuously improved to keep pace with cybercriminals and adapt its capabilities over time.
Below are key ways in which cybersecurity AI is being improved.
1. Better training for AI models
AI models are getting better training thanks to increased computation and training data size. As these models ingest greater amounts of data, they have more examples to learn from and can draw more accurate and nuanced conclusions from the examples it is shown.
As a result, cybersecurity AI tools are better at identifying patterns and anomalies in large datasets and learning from past incidents, which enables them to more accurately predict potential threats, among other cybersecurity use cases.
2. Advances in language processing technology
Thanks to increases in data resources and computing power, language processing technology has made significant advances in the past few years. These advances, including enhanced capabilities to learn from complex and context-sensitive data, will significantly improve cybersecurity AI tools that automatically generate step-by-step remediation instructions, threat intelligence, and other code or text.
3. Threat intelligence integration
Cybersecurity AI systems are being enhanced by integrating with threat intelligence feeds. This enables them to stay updated on the latest threat information and adjust their defenses accordingly.
4. Deep learning
A subset of machine learning, deep learning is a neural network with three or more layers. Simulating the behavior of the human brain, these neural networks attempt to learn from large amounts of data and make more accurate predictions than a neural network with a single layer.
Due to its ability to process vast amounts of data and recognize complex patterns, deep learning technology is helping contribute to more accurate threat hunting, management, and response.
5. More resources for AI development and use
As AI development and usage continue to skyrocket in cybersecurity and other industries, governments and other authoritative bodies like NIST, CISA, and OWASP are publishing resources to help individuals and businesses manage the risks while leveraging the benefits. These resources will help provide developers with best practices for improving AI in cybersecurity and beyond. Some examples include:
Recommended reading
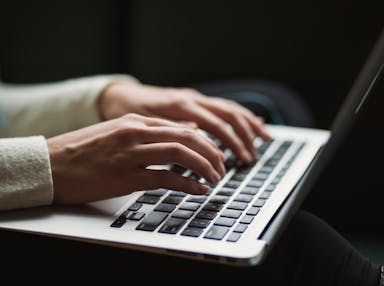
190 Cybersecurity Statistics to Inspire Action This Year
Harnessing the power of AI in your cybersecurity strategy
AI is increasingly being used in cybersecurity to reap a range of benefits, from improving the efficiency of cybersecurity professionals to identifying and responding to critical threats in less time.
Secureframe is committed to simplifying security and compliance with AI. Its latest AI innovations include:
- Comply AI for Remediation provides AI-powered remediation guidance to help speed up cloud remediation and time-to-compliance.
- Comply AI for Risk automates the risk assessment process to save you time and reduce the costs of maintaining a strong risk management program.
- Comply AI for Policies leverages generative AI so you can save hours writing and refining policies.
- Secureframe’s questionnaire automation leverages AI so you can quickly answer security questionnaires and RFPs with more than 90% accuracy.
To learn more about how Secureframe uses automation and AI to simplify security and compliance, schedule a demo.
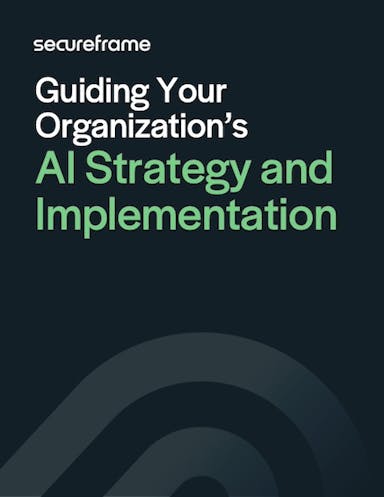
Guiding Your Organization's AI Strategy and Implementation
Follow these best practices to effectively implement AI while addressing concerns related to transparency, privacy, and security.
FAQs
How is AI being used in cybersecurity?
AI is being used in cybersecurity to supplement the efforts of human experts by automating tasks that are highly repetitive, manually-intensive, and tedious for them to complete. Since AI can analyze massive volumes of data, identify patterns that humans might miss, and adapt and improve its capabilities over time, it excels at threat detection, threat management, threat response, endpoint security, and behavior-based security.
What is responsible AI in cyber security?
Responsible AI in cybersecurity refers to the design and deployment of safe, secure, and trustworthy artificial intelligence in the industry. The goal is to increase transparency and reduce risks like AI bias by promoting the adoption of specific best practices, such as red-team testing.
How are AI and machine learning changing the cybersecurity landscape?
AI and machine learning are being used to strengthen cybersecurity in unprecedented ways, like flagging concealed anomalies, identifying attack vectors, and automatically responding to security incidents. They are also being used to launch increasingly sophisticated and frequent cyber attacks as well.