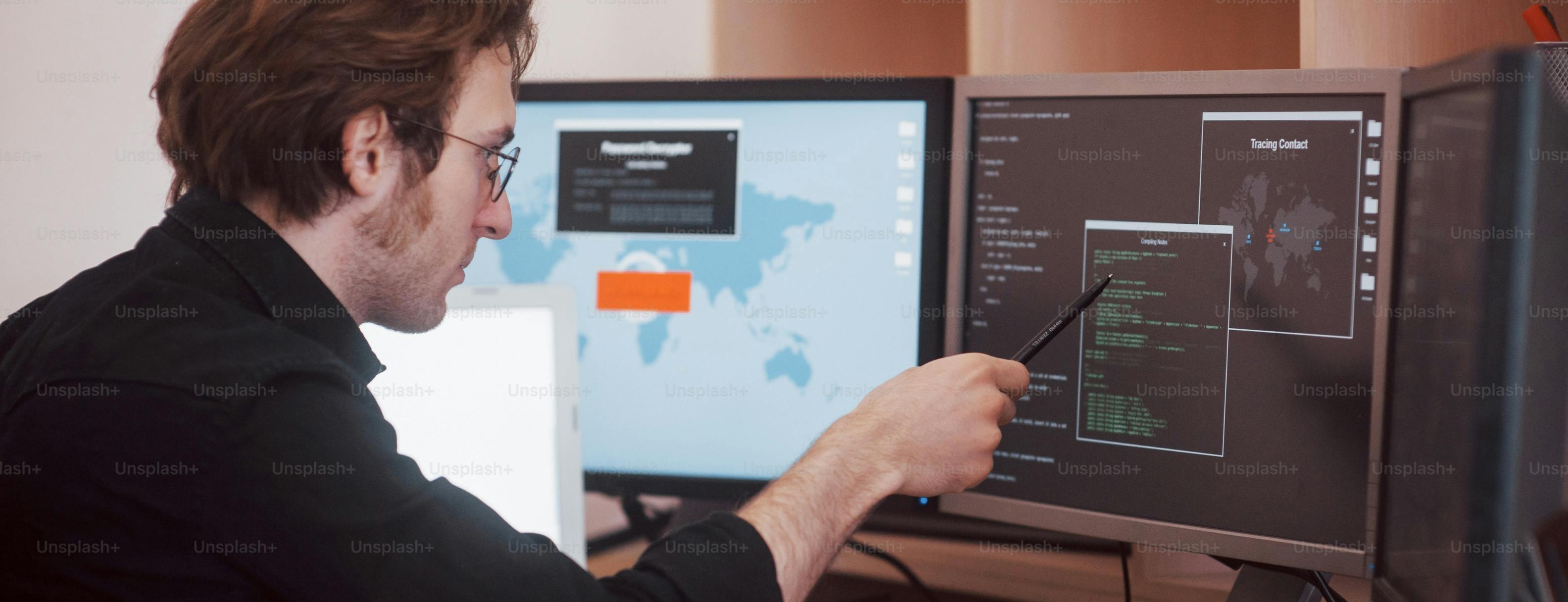
How Artificial Intelligence Will Affect Cybersecurity in 2024 & Beyond
Emily Bonnie
Senior Content Marketing Manager
Rob Gutierrez
Senior Cybersecurity and Compliance Manager, CISA, CCSK, CMMC RP
AI has already begun affecting the future of cybersecurity.
Today, malicious actors are manipulating ChatGPT to generate malware, pinpoint vulnerabilities in code, and bypass user access controls. Social engineers are leveraging AI to launch more precise and convincing phishing schemes and deepfakes. Hackers are using AI-supported password guessing and CAPTCHA cracking to gain unauthorized access to sensitive data.
In fact, 85% of security professionals that witnessed an increase in cyber attacks over the past 12 months attribute the rise to bad actors using generative AI.
Yet AI, machine learning, predictive analytics, and natural language processing are also being used to strengthen cybersecurity in unprecedented ways — flagging concealed anomalies, identifying attack vectors, and automatically responding to security incidents.
As a result of these advantages, 82% of IT decision-makers plan to invest in AI-driven cybersecurity in the next two years and almost half (48%) plan to invest before the end of 2023.
To fully grasp the impact of AI in cybersecurity, CISOs and other security and IT leaders must understand the benefits and risks of artificial intelligence. We’ll take a closer look at these below.
The advantages of AI in cybersecurity
Despite headlines being dominated by weaponized AI, artificial intelligence is a powerful tool for organizations to enhance their security posture. Algorithms capable of analyzing massive amounts of data make it possible to quickly identify threats and vulnerabilities, mitigate risks, and prevent attacks. Let’s take a closer look at these use cases.

1. Identifying attack precursors
AI algorithms, particularly ML and deep learning models, can analyze massive volumes of data and identify patterns that human analysts might miss. This ability will facilitate early detection of threats and anomalies, preventing security breaches and allowing systems to become proactive rather than reactive in threat hunting.
AI systems can be trained to run pattern recognition and detect ransomware or malware attacks before they enter the system.
Predictive intelligence paired with natural language processing can scrape news, articles, and studies on emerging cyber threats and cyberattack trends to curate new data, improve functionality, and mitigate risks before they materialize into full-scale attacks.
2. Enhancing threat intelligence
Generative AI, a type of AI that uses deep-learning models or algorithms to automatically create text, photos, videos, code, and other output based on the datasets they are trained on, can not only help analysts identify potential threats but also understand them better.
Previously without AI, analysts had to use complex query languages, operations, and reverse engineering to analyze vast amounts of data to understand threats. Generative AI algorithms can automatically scan code and network traffic for threats and provide rich insights that help analysts understand the behavior of malicious scripts and other threats.
Recommended reading
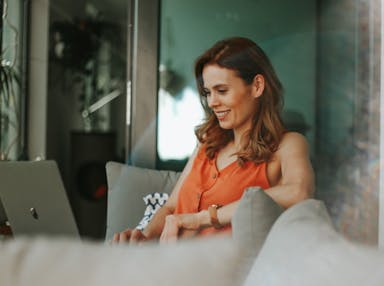
Generative AI in Cybersecurity: How It’s Being Used + 8 Examples
3. Strengthening access control and password practices
AI enhances access control and password practices by employing advanced authentication mechanisms. Biometric authentication such as facial recognition or fingerprint scanning can strengthen security measures by reducing reliance on traditional passwords.
AI algorithms can also analyze login patterns and behaviors to identify minor behavioral anomalies and suspicious login attempts, allowing organizations to mitigate insider threats and address potential security breaches faster.
4. Minimizing and prioritizing risks
The attack surface for modern enterprises is massive, and growing every day. The ability to analyze, maintain, and improve such a significant vulnerability landscape now requires more than humans alone can reasonably achieve.
As threat actors capitalize on emerging technologies to launch progressively sophisticated attacks, traditional software and manual techniques simply can’t keep up.
Artificial intelligence and machine learning are quickly becoming essential tools for information security teams to minimize breach risk and bolster security by identifying vulnerabilities in systems and networks. Machine learning models can scan infrastructure, code, and configurations to uncover weaknesses that could be exploited by attackers. By proactively identifying and patching vulnerabilities, organizations can significantly reduce the risk of successful cyberattacks.
By leveraging machine learning algorithms, organizations can automate risk assessments and allocate resources effectively. AI can provide insights into the likelihood and consequences of different types of attacks, enabling cybersecurity teams to prioritize mitigation efforts efficiently.
In other words, AI-based cybersecurity systems can prioritize risks based not only on what cybercriminals could use to attack your systems, but on what they’re most likely to use to attack your systems. Security and IT leadership can better prioritize and allocate resources to the highest vulnerabilities.
5. Automating threat detection and response
With AI, cybersecurity systems can not only identify but also respond to threats automatically.
- Malicious IP addresses can be blocked automatically
- Compromised systems or user accounts can be shut down immediately
- ML algorithms can analyze emails and web pages to identify and block potential phishing attempts
AI-powered systems automate threat detection processes, providing real-time monitoring and rapid response times. Machine learning algorithms continuously analyze network traffic, user behavior, and system logs to identify suspicious activities.
By leveraging AI's ability to process and analyze massive volumes of data, organizations can detect and respond to threats immediately, minimizing the time window for attackers to exploit vulnerabilities.
Recommended reading
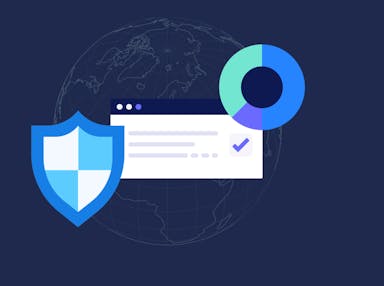
6 Benefits of Continuous Monitoring for Cybersecurity
Intelligent algorithms can analyze security alerts, correlate events, and provide insights to support decision-making during an incident. AI-powered incident response platforms can automate investigation workflows, rapidly identify the root cause of an incident, and suggest appropriate remedial actions. These capabilities empower security teams to respond quickly, minimizing the impact of security breaches.

6. Increasing human efficiency & effectiveness
82% of data breaches involve human error. By automating routine manual tasks, AI can play a pivotal role in reducing the likelihood of misconfigurations, accidental data leaks, and other inadvertent mistakes that could compromise security.
AI also equips cybersecurity teams with powerful tools and insights that improve their efficiency and effectiveness. Machine learning models can analyze vast amounts of threat intelligence data, helping teams more fully understand the threat landscape and stay ahead of emerging threats.
AI-powered security and compliance automation platforms streamline workflows, enabling teams to respond to incidents faster and with greater precision. By offloading time-consuming manual tasks, cybersecurity professionals can focus on strategic initiatives and higher-level threat analysis.
From predictive analytics to automated threat detection and incident response, AI augments the capabilities of cybersecurity teams, enabling proactive defense measures. Embracing AI technology empowers organizations to stay ahead in the cybersecurity landscape and safeguard their valuable assets.

The disadvantages of AI in cybersecurity
Cybersecurity leaders that want to implement AI to enhance their security posture must address a range of challenges and risks first, including those related to transparency, privacy, and security.

Data privacy concerns
AI systems often require large amounts of data, which can pose privacy risks. If AI is used for user behavior analytics, for example, it may need access to sensitive personal data.
Where does AI data reside? Who can access it? What happens when the data is no longer needed? More companies are walking a tightrope to balance user privacy with data utility.
Proper AI governance is foundational to minimizing financial and reputational risk. Over the coming years, there will be an increased demand for effective ways to monitor AI performance, detect stale models or biased results, and make the proper adjustments.
Organizations will need to adopt an AI governance approach that encompasses the entire data lifecycle, from data collection to processing, access, and disposal. Privacy by design will need to become a greater focus in the AI lifecycle and in AI governance strategies, including data anonymization techniques that preserve user privacy without impacting data’s usefulness for AI applications.
Reliability and accuracy
While AI systems can process vast amounts of data quickly, they are not perfect. False positives and negatives can occur, potentially leading to wasted efforts and time or overlooked threats.
Since AI and ML algorithms are only as good as the data they ingest, organizations will need to invest in data preparation processes to organize and clean data sets to ensure reliability and accuracy.
This is increasingly important as data poisoning becomes more prevalent. Data poisoning involves adding or manipulating the training data of a predictive AI model in order to affect the output. In a landmark research study, injecting 8% of “poisonous” or erroneous training data was shown to decrease AI’s accuracy by as much as 75%.
Lack of transparency
AI systems, especially deep learning models, often function as black boxes, making it challenging to understand how they arrive at specific decisions or predictions. This lack of transparency creates a barrier for cybersecurity experts who need to understand the reasoning behind an AI system's outputs, particularly when it comes to identifying and mitigating security threats. Without transparency, it becomes difficult to trust the decisions made by AI systems and validate their accuracy.
In addition, AI systems may generate false positives, overwhelming security teams in constantly putting out fires. False negatives can result in missed threats and compromised security. Lack of transparency into the reasons for these errors makes it difficult to fine-tune AI models, improve accuracy, and rectify any real issues. Cybersecurity experts need to be able to understand and validate the decisions made by AI systems to effectively defend against evolving cyber threats.
Training data and algorithm bias
There are different types of bias that may affect an AI system. Two key ones are training data and algorithmic bias. Let’s take a closer look at them below.
Training data bias
When the data used to train AI and machine learning (ML) algorithms is not diverse or representative of the entire threat landscape, the algorithms may make mistakes, like overlook certain threats or identify benign behavior as malicious. This is often the result of bias in the AI developers that created the training data set.
For example, say an AI developer believed that hackers from Russia were the biggest threat to US companies. As a result, the AI model would be trained on data skewed toward threats from this one geographical region and might overlook threats originating from different regions, particularly domestic threats.
The same would be true if the AI developer believed that one attack vector, like social engineering attacks, was more prevalent than any other. As a result, the AI model may be effective against this attack vector but fail to detect other prominent threat types, like credential theft or vulnerability exploits.
Algorithmic bias
The AI algorithms themselves can also introduce bias. For example, say a system uses pattern matching to detect threats. It may raise false positives when a benign activity matches a pattern, like flagging any email containing abbreviations or slang as potential phishing attacks. An algorithm that favors false positives in this way can lead to alert fatigue. An AI system that uses pattern matching may conversely fail to detect subtle variations in known threats, which can lead to false negatives and missed threats.
If unaddressed, both types of bias can result in a false sense of safety, inaccurate threat detection, alert fatigue, vulnerability to new and evolving threats, and legal and regulatory risk.
Recommended reading
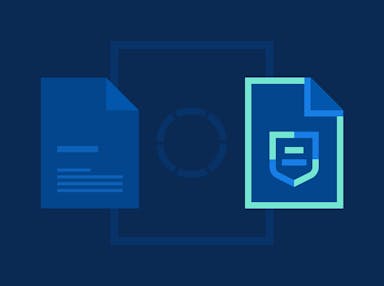
Respond to Security Questionnaires and RFPs Quickly and Accurately with Artificial Intelligence
How cybersecurity leaders can successfully incorporate AI into their security programs
As the use of AI in cybersecurity continues to grow, CISOs and other cybersecurity leaders will play a critical role in harnessing the potential of AI while ensuring its secure and effective implementation. By following these best practices, these leaders can effectively implement AI while addressing concerns related to transparency, privacy, and security.
1. Align AI strategy with business & security objectives
Before embarking on AI implementation, cybersecurity leaders must align AI strategy with the organization's broader business and security objectives. Clearly define the desired outcomes, identify the specific cybersecurity challenges AI can address, and ensure that AI initiatives align with the organization's overall security strategy.
2. Invest in skilled AI talent
While AI can significantly enhance a cybersecurity system, it should not replace human expertise. Building an AI-ready cybersecurity team is crucial.
Invest in recruiting information security professionals who understand AI technologies. By having a team with the right expertise, you can effectively evaluate AI solutions, implement them, and continuously optimize their performance. Cybersecurity leaders should promote AI literacy within their organizations to help team members use AI tools effectively and understand their limitations.
3. Thoroughly evaluate AI solutions
Take a diligent approach when evaluating AI solutions. Assess the vendor's reputation, the robustness of their AI models, and their commitment to cybersecurity and data privacy. Conduct thorough proof-of-concept trials and evaluate how well the solution integrates with existing cybersecurity infrastructure. Ensure that the AI solution aligns with your organization's security requirements and regulatory obligations.
You should also evaluate the preventative measures they take to minimize bias in their solutions. Employing robust data collection and preprocessing practices, having diversity on AI developer and deployment teams, investing in continuous monitoring, and employing multiple layers of AI are just a few ways to mitigate bias to maximize the potential and effectiveness of AI in cybersecurity.
4. Establish a robust data governance framework
AI relies on high-quality, diverse, and well-curated data. Establish a robust data governance framework that ensures data quality, integrity, and privacy. Develop processes for collecting, storing, and labeling data while adhering to relevant regulations. Implement measures to protect data throughout its lifecycle and maintain strict access controls to safeguard sensitive information.
Finally, choose AI models that are explainable, interpretable, and can provide insights into their decision-making processes.
5. Implement strong security measures for AI infrastructure
Ensure the security of AI infrastructure by implementing robust security measures. Apply encryption to sensitive AI model parameters and data during training, deployment, and inference. Protect AI systems from unauthorized access and tampering by implementing strong authentication mechanisms, secure APIs, and access controls. Regularly patch and update AI frameworks and dependencies to address security vulnerabilities.
Recommended reading
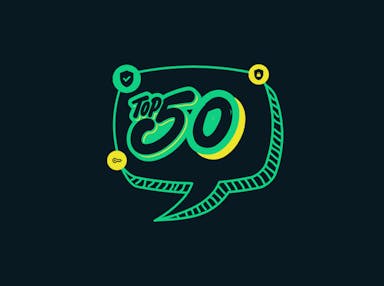
50 Influential CISOs and Cybersecurity Leaders to Follow
2024 AI Cybersecurity Checklist
Download this checklist for more step-by-step guidance on how you can harness the potential of AI in your cybersecurity program in 2024 and beyond.

How Secureframe is embracing the future of AI in cybersecurity
Artificial intelligence is set to play an increasingly pivotal role in cybersecurity, with the potential to empower IT and infosec professionals, drive progress, and improve information security practices for organizations of all sizes.
Secureframe is continuing to launch new AI capabilities to help customers automate tasks related to security, risk, and compliance. The latest AI innovations include:
- Comply AI for remediation: Improve the ease and speed of fixing failing controls in your cloud environment to improve test pass rate and get audit ready.
- Comply AI for risk: Automate the risk assessment process to save time and resources and improve your risk awareness and response.
- Comply AI for policies: Leverage generative AI to save hours writing and refining policies.
- Questionnaire automation: Use machine learning-powered automation to save hundreds of hours answering RFPs and security questionnaires.
Use trust to accelerate growth
FAQs
Will AI take over cybersecurity?
No, AI will not completely take over cybersecurity. Other technologies (like behavioral biometrics, blockchain, and quantum mechanics) will remain prevalent and human expertise will remain critical for more complex decision-making and problem-solving, including how to develop, train, deploy, and secure AI effectively and ethically. However, AI will likely lead to new cybersecurity solutions and careers.
Can AI predict cyber attacks?
Yes, AI can help predict cyber attacks by monitoring network traffic and system logins to identify unusual patterns that may indicate malicious activities and threat actors. In order to do so effectively, the AI model must be trained on a large data set that comprehensively represents the threat landscape now and as it evolves.
What is an example of AI in cybersecurity?
An example of AI in cybersecurity is automated cloud remediation. For example, if a test is failing in the Secureframe platform, Comply AI for Remediation can quickly generate initial remediation guidance as code so users can easily correct the underlying configuration or issue causing the failing test in their cloud environment. This ensures they have the appropriate controls in place to meet information security requirements.