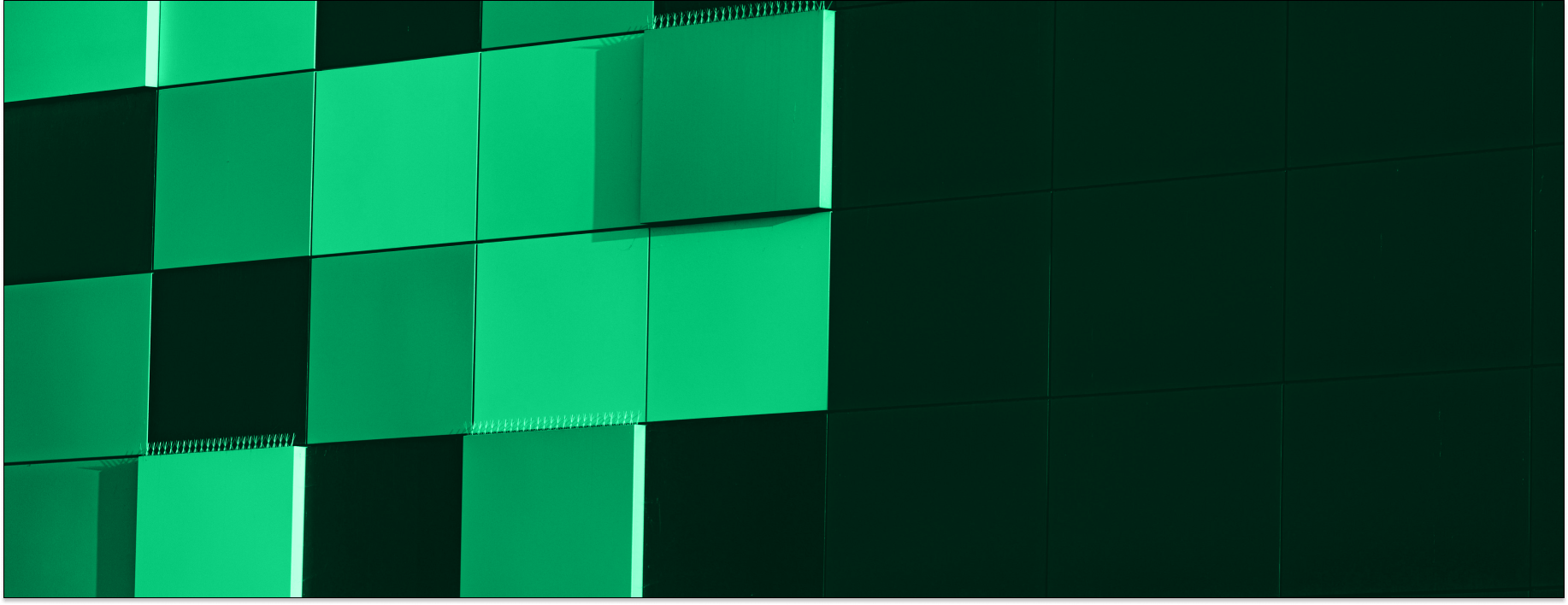
Navigating AI Risks: Conducting Risk Assessments for High, Limited, and Minimal-Risk AI
Kyle McLaughlin
General Counsel
Anna Fitzgerald
Senior Content Marketing Manager
The rapid proliferation of artificial intelligence (AI) over the past few years has led to a significant increase in innovation, while also creating uncertainty over how this technology affects how we think about governance, risk, and compliance.
In its simplest implementation, AI has the ability to tackle repetitive, time-consuming, mundane tasks with minimal human intervention. At the same time, AI is powerful enough to conduct more complex activities like helping make faster and more accurate healthcare decisions targeting personalized treatment plans, filtering thousands of employment applications to determine suitability for a job, detecting potential fraud, and determining credit worthiness related to worldwide financial transactions. The latter scenarios tend to raise concerns about AI, most often related to decision-making in place of humans, along with privacy and security risks.
While these may seem like new issues, most of these concerns can be addressed with the same due diligence and risk mitigation activities that organizations have been performing for years.
Recommended reading
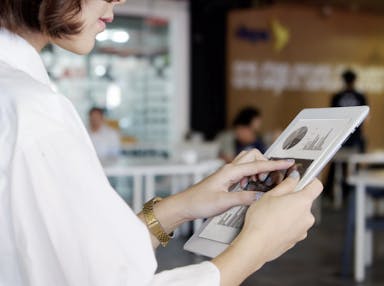
Risk and Compliance in the Age of AI: Challenges and Opportunities
The challenge with assessing AI risk
Fears about AI are often amplified in the absence of a clear understanding of how AI works and the extent of its capabilities. While current iterations of AI are powerful, it is not an omnipotent technology. Just as underestimating AI’s capabilities would be a mistake, overestimating its capabilities can lead to unnecessary panic or concern.
Today, AI’s decision-making is still largely dependent on the data that is used to train the AI model, which is more limited (and often biased) than most people realize. Additionally, many AI systems are designed with human oversight and intervention in mind, especially in the B2B world where it would be unwise to have a nascent system making independent decisions on behalf of an organization’s employees, customers, vendors, and systems.
Large corporations typically have large teams of people dedicated to assessing the risk of not only their internal development and implementation of AI systems, but those systems developed by their vendors and partners as well. While small businesses and start-ups may not have the same resources or time to spend on review of internal and external AI systems, it is still critical to perform an appropriate risk assessment of these systems.
But what should these companies be primarily concerned about when it comes to AI? What is considered “scary” AI that warrants a deep dive? When can an AI review be limited to traditional privacy and security considerations? How should we account for training of AI models?
The European Union AI Act provides the first regulatory roadmap that helps answer these questions.
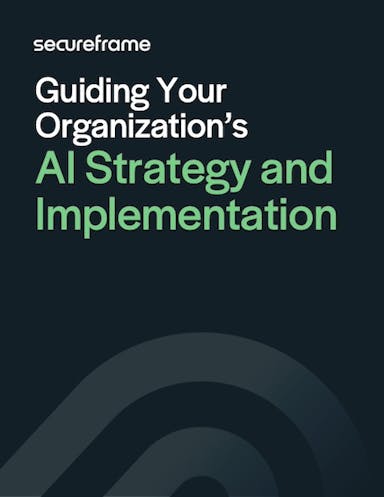
Guiding Your Organization's AI Strategy and Implementation
Follow these best practices to effectively implement AI while addressing concerns related to transparency, privacy, and security.
How the EU AI Act can help guide AI risk assessments
The AI Act applies to providers and users of AI systems in the EU, regardless of whether they are established within the EU. It also covers AI systems available or used within the EU market. The AI Act aims to ensure that AI systems are safe and respect fundamental rights and values, including privacy, non-discrimination, and human dignity.
The AI Act categorizes AI systems into four risk levels, with different rules for each level:
- Unacceptable Risk: AI systems that pose a clear threat to safety, livelihoods, and rights are prohibited (e.g. cognitive behavioral manipulation or social scoring by governments).
- High Risk: AI systems used in critical areas such as healthcare, law enforcement, transportation, and employment, or other areas where there is a significant risk of harm to people’s health, safety or fundamental rights are considered high risk. These systems must comply with stringent AI-specific requirements covering risk management, data governance, and transparency.
- Limited Risk: AI systems with limited risk are those that pose little to no threat to people’s safety, privacy, or fundamental rights. These systems do not carry stringent AI-specific reviews beyond the standard risk, privacy and security measures that should be considered when implementing any technology. However, these limited risk activities must still meet transparency obligations, such as informing users they are interacting with an AI system.
- Minimal Risk: AI systems with minimal risk, like spam filters or video games, are largely unregulated, but are still subject to general safety and privacy transparency rules.
Although these categories are somewhat broad, they help establish a logical framework for how companies large and small should think about their AI risk assessments. Given that B2B companies should never be engaging in prohibited AI activities, we’ll focus on High and Limited Risk activities.
Some examples of High Risk activities include:
- Credit scoring
- Evaluating employment applications
- Healthcare implementations
- Apps that scan biometric features such as those that allow a user to virtually try on clothing or other apparel
- Transportation safety (e.g. autonomous driving)
Limited Risk activities can include:
- Systems that provide AI-generated content in response to a request where the likelihood of harm to individuals is not significant (e.g. chatbots or similar AI-assist features)
- AI-powered search engines
- Deep fake content
When assessing the impact of AI systems on a business’ internal environment and those of its customers and partners, the considerations afforded to Limited and Minimal Risk activities are ultimately very similar to traditional data privacy and security risks. As with any other technology, it is important to determine the following:
- What data is at play (especially if any customer or other third-party data will be processed by the AI system)
- How that information is used, stored, and secured
- Why that particular level of data is necessary in order to perform the desired output
- Whether any of the data involved is confidential or contains personal data (input or output)
- Whether and how the AI system will interact with other internal or external applications or systems
- Whether and how internal production environments may be impacted, etc.
In addition to these traditional considerations, Limited Risk AI systems must include transparent notices that make clear to the user that they are interacting with an AI system or receiving AI-generated content. Such notices must be in machine-readable format and detectable as AI-generated or manipulated.
Recommended reading
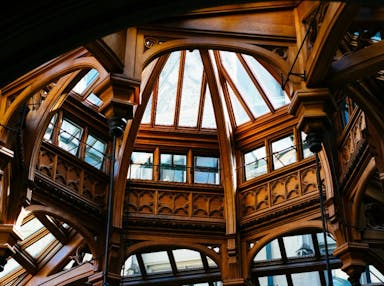
Comparing AI Frameworks: How to Decide If You Need One and Which One to Choose
AI risk assessments for Limited and Minimal Risk AI activities
Due to the nature of their classification, most Limited and Minimal Risk AI activities involve situations where an output is generated and provided to a human for any next steps, rather than true automated decision-making with implementation. As a result, the output of these AI systems is generally gated by humans.
But what about the input? How should we assess training of AI algorithms and related machine learning techniques? In most cases, the answer is once again similar to traditional data privacy, confidentiality, and security considerations.
For well over a decade, SaaS and cloud-based software companies have relied on some combination of customer data and “usage” data (meaning data related to a customer’s use and interaction with the cloud software) to deliver, build, and improve such software. Even those companies that don’t claim to use AI generally have clauses in their terms of use that state that they can use some form of usage or analytics data to improve their software.
If these companies did not have this data usage right, the economies of scale related to providing a cloud-based, one-to-many software delivery model would become untenable. Each customer’s environment would become not only logically isolated (as is common with cloud software), but issues with one entity’s software instance may not be able to be used to identify similar events in other instances. Feature usage and development statistics would similarly only be applicable to one customer, instead of permitting cross-customer determinations around sunsetting or future feature advancements.
Whether and how data is used to train AI algorithms is not a significantly different assessment exercise than the one used for traditional usage or analytics data rights. However, there are some important distinctions.
Any data that is used as an input to train a particular AI algorithm can, in theory, be produced as an output of that AI system. While it may be helpful for an AI system to understand generalized commonalities across different businesses and use cases, getting too specific may result in the cross-sharing of confidential information.
As it relates to confidential, sensitive, or personal data, it’s a best practice to ensure that this data is not used to train AI models that are accessible by untrusted third parties or any personnel who should not have access to such data. Similarly, interactions between AI training models and intellectual property deserves additional scrutiny to ensure that the AI system will not use, modify, reverse engineer, or otherwise distribute such intellectual property in a manner that does not protect the author’s rights.
AI risk assessments for High Risk Activities
High Risk activities require a more robust assessment since, by their nature, these activities involve a significant risk of harm to people’s health, safety, or fundamental rights. As a result, errors or biases related to these activities can have severe consequences. The most critical area of focus for High Risk activities involve AI systems that can make decisions or implement outcomes without the gating actions of a human (e.g., automated decision-making or profiling).
In addition to the requirements set forth in the AI Act, these activities may also be covered by various privacy and product safety laws. For example, even where a human is part of the process, the GDPR still requires that notice and an appeals process be made available to individuals whose personal data is processed by an AI system in the course of making any determination that affects such individual’s legal rights or that has a similar impact on their circumstances, behavior, or opportunities (e.g., credit applications or employment-related scoring). Moreover, to the extent an AI system is making decisions or implementing outcomes without the gating actions of a human, the system (or more appropriately the developer or back-end user of the system) could be considered a data controller under the GDPR.
When developing or utilizing a third-party system that involves High Risk activities, it is critical to ensure that an appropriate risk management system is implemented throughout the lifecycle of the corresponding AI system. This includes, among other considerations:
- Ensuring proper data governance through sufficient high-quality data sets;
- Recording detailed technical information as to how the AI system operates;
- Ensuring transparency through clear and concise communication regarding the capabilities and instructions for use of the AI system;
- Providing human oversight allowing for the monitoring and intervention of the AI system where appropriate; and
- Conducting a security risk assessment and implementing security mechanisms that take into account the state of the art to protect the AI system and corresponding data.
When examining the final bullet point above, organizations should refrain from making determinations in a vacuum regarding what is considered state of the art. Increasingly, global privacy, security, and now AI regulations require organizations to implement, at minimum, industry standard security and compliance measures.
There are a variety of industry frameworks that address these measures and make recommendations or provide guidelines for implementing industry standard or best-in-class practices.
While unique innovations in security technology should always be considered, implementing established frameworks such as CIS Controls, appropriate ISO and NIST frameworks, Essential Eight, Cyber Essentials and upcoming EU Harmonised Standards (to name a few) is the most compelling method of meeting accepted governance, risk, and compliance standards.
Recommended reading
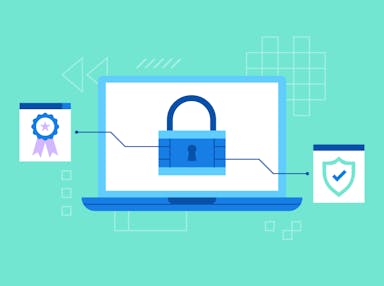
Essential Guide to Security Frameworks & 14 Examples
Using your existing GRC strategy for AI
After diving into the analysis and classification of AI systems by risk level, it becomes evident that the related governance, risk, and compliance considerations are only incrementally unique at this stage.
Start with asking the same questions and performing the same diligence routinely performed with any software or third-party that may have access to confidential, sensitive, or personal data, and pay attention to what the AI system’s actual capabilities are, how such capabilities are trained and/or managed, and whether clear and open disclosures are available throughout a user’s interaction with such system.
In all cases, it is best to ensure that an appropriate industry standard security framework is used to guide the implementation of governance, risk and compliance measures inherent to any AI system.
***This blog post does not constitute legal advice and should not be relied upon as a legal opinion. Please consult appropriate legal, compliance and security professionals prior to making any assessments.***