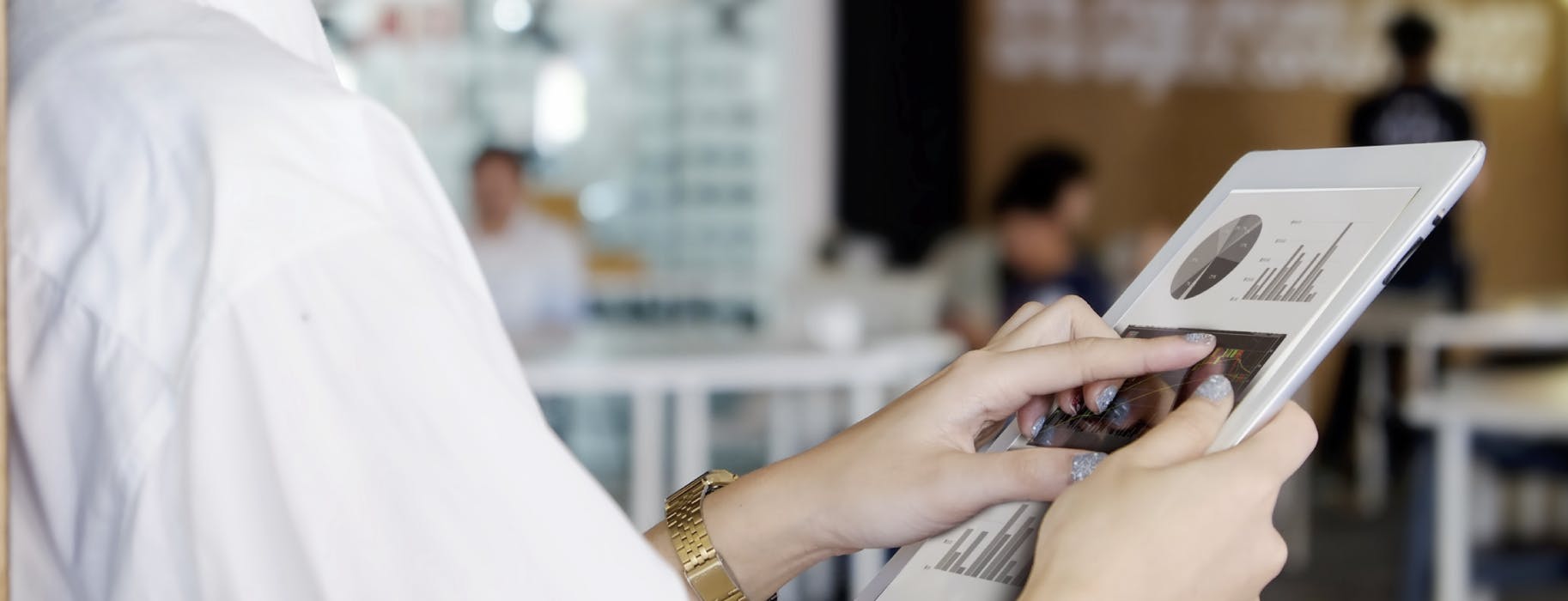
Risk and Compliance in the Age of AI: Challenges and Opportunities
Advances in artificial intelligence are a double-edged sword. While AI and machine learning technologies are being used to improve cybersecurity in powerful ways, many organizations struggle to understand exactly how the use of AI tools impacts their risk profile, attack surface, and compliance posture.
Misuse of AI solutions can raise significant data privacy concerns, introduce bias in the strategic decision-making process, lead to non-compliance violations, and increase third-party risks.
Below, we’ll unpack the current risks of AI tools, explore how different forms of AI and ML are being used to enhance risk management and improve security compliance, and share key steps organizations can take today to step confidently into the age of AI.
Top AI risks and challenges facing organizations in 2024
The integration of AI into business operations is a game-changer for many organizations, offering unparalleled opportunities for growth and innovation. While AI brings significant opportunities for efficiency, it also introduces a range of risks for organizations to navigate.

AI risk management challenges
Since the release of ChatGPT 4 in March 2023, the widespread adoption of AI is skyrocketing. Yet efforts to manage the associated risks of AI tools are lagging.
93% of organizations surveyed say they understand generative AI introduces risk, but only 9% say they’re prepared to manage those threats. Another study found that one-fifth of organizations that use third-party AI tools don’t evaluate their risks at all.
Organizations are faced with the difficult choice of either falling behind competitors who are adopting innovative AI solutions, or exposing themselves to a host of risks — including data breaches, reputational damage, regulatory penalties, and compliance challenges.
Introduction of new vulnerabilities
The use of AI tools can introduce security vulnerabilities that can easily go undetected and unresolved.
For example, many developers have turned to generative AI tools to increase efficiency. But a Stanford study found that software engineers who use code-generating AI systems are more likely to introduce security vulnerabilities in the apps they develop. A similar study that assessed the security of code generated by GitHub Copilot found that nearly 40% of AI suggestions led to code vulnerabilities.
AI tools can also introduce risk to intellectual property and other sensitive data. Any IP or customer data that’s fed to the tool could also be stored or accessed by other service providers. And because data entered into generative AI tool prompts can also become part of its training set, any sensitive information inputs could end up in outputs for other users. This may seem like a low-risk scenario, but a recent study by Cyberhaven found that 11% of the data employees paste into ChatGPT is confidential.
AI models are only as good as the data they’re fed, making data poisoning another significant risk. If AI models can be tricked, they could execute malware or bypass security controls to give malware stronger access privileges.
Data privacy concerns
AI systems require huge volumes of data, posing significant data privacy risks. AI models that are used to analyze customer or user behavior, for example, may need access to sensitive personal information. GenAI tools may also share user data with third parties and service providers, potentially violating data privacy laws. Regulation has already been implemented in the EU and China, with proposed regulations in the US, UK, Canada, and India.
Organizations will need to emphasize AI data privacy in their data governance, including data anonymization techniques that preserve user privacy without impacting data utility. Proper AI governance will also help monitor AI performance, detect stale models, and identify bias.
Potential for bias
When data sets used to train AI and machine learning algorithms are not diverse or comprehensive enough, it can negatively impact the AI model’s performance. Threats can be overlooked, or benign behavior can be identified as malicious.
Imagine an AI-based Intrusion Detection System that’s trained primarily on a dataset of the most common recent cyberattacks, such as malware or ransomware attacks.
This AI system might be highly efficient at detecting similar types of attacks in the future. But as the cyber threat landscape evolves and new attacks emerge, the system may fail to recognize and respond to these threats.
The bias is towards known types of cyber threats and against newer, evolving threats — potentially leading to vulnerabilities in the network or system the algorithm is supposed to secure.
Algorithmic bias also poses a significant risk. For instance, pattern recognition used in threat detection might incorrectly flag harmless activities, such as typos or slang in emails, as phishing threats. Too many of these false positives can lead to alert fatigue.
Regulatory and compliance risk
Policymakers worldwide are grappling with the significant ethical and practical issues brought up by the potential use and misuse of AI technologies. They’re challenged to strike a balance between fostering innovation and keeping pace on a global stage with protecting both individuals and society at large from existential risks.
Because the AI industry is still in its infancy, legislation is likely to be drafted based on current industry leaders — but it’s not obvious which AI technologies will be most successful or which players will become dominant in the industry.
Some governments and regulatory bodies are taking a wait-and-see approach, while others are proactively proposing or enacting legislation to regulate the development and use of AI.
The EU Artificial Intelligence Act is currently in provisional agreement, likely coming into effect in 2025. In the US, Biden’s Executive Order on the Safe, Secure, and Trustworthy Development and Use of Artificial Intelligence established the U.S. Artificial Intelligence Safety Institute (USAISI), which will lead efforts to develop standards for the safety, security, and testing of advanced AI models. In the absence of sweeping federal legislation, expect to see industry-specific agency actions across healthcare, financial services, housing, workforce, and child safety sectors, as well as additional executive orders.
The increasing adoption of AI tools and their impact on organizational risk has also led to the development of several new AI security frameworks. For example, ISO 42001 offers a broad approach to information security that applies to AI systems, while NIST AI RMF provides comprehensive guidelines for managing risks specifically associated with AI technologies. Evaluating and adopting these frameworks can help organizations navigate the complex landscape of AI risks and implement AI solutions securely and responsibly.
2024 is shaping up to be a pivotal year for both AI development and regulatory changes. With so much in flux, organizations and compliance professionals are struggling to understand how emerging regulatory requirements will impact their operations and compliance programs.
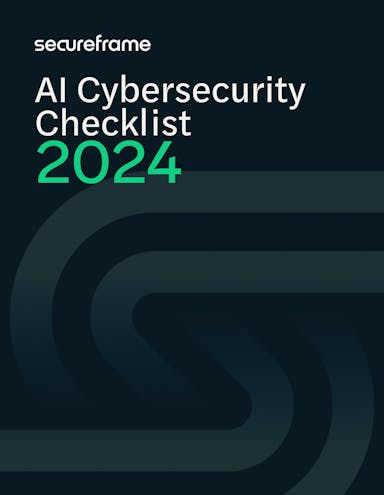
2024 AI Cybersecurity Checklist
Download this checklist for step-by-step guidance on how you can effectively implement AI while addressing concerns related to transparency, privacy, and security.
How artificial intelligence is enhancing risk management
AI's ability to process and analyze vast amounts of data, along with its predictive capabilities, makes it a powerful tool in risk management. It helps organizations anticipate, identify, and mitigate risks more effectively, leading to better decision-making and more resilient business operations.
Here are a few significant use cases for AI in risk management:
- Risk identification through predictive analytics: AI algorithms excel at identifying patterns and trends in large datasets. These predictive analytics allow organizations to foresee potential risks such as market fluctuations, supply chain risks, or operational failures before they materialize. AI can also model various risk scenarios and predict their outcomes, helping managers make informed decisions about risk mitigation tactics.
- Automated risk assessment workflows: AI can automate the time-consuming and complex risk assessment process, helping organizations quickly identify risk factors and prioritize based on potential impact. Advanced tools such as Secureframe’s Comply AI can also suggest risk treatment plans based on the organization’s specific security posture and risk profile.
- Real-time risk monitoring: Because AI systems can analyze data in real time, organizations get immediate insights into potential risks. AI can detect cybersecurity anomalies that may indicate a breach, and in financial services, continuous monitoring allows for fraud detection and financial crime prevention.
- Scenario analysis and stress testing: AI can simulate a range of adverse scenarios and stress test an organization’s resilience to various risks, from financial downturns to operational disruptions. Organizations can then better plan and prepare for potential crises.
- Third-party and supply chain risk management: AI tools can analyze global supply chain networks, predict disruptions due to natural disasters or geopolitical issues, and suggest mitigation strategies to minimize the impact on operations. They can also analyze third-party contracts to highlight risks such as unfavorable terms, hidden costs, or clauses that might pose a liability.

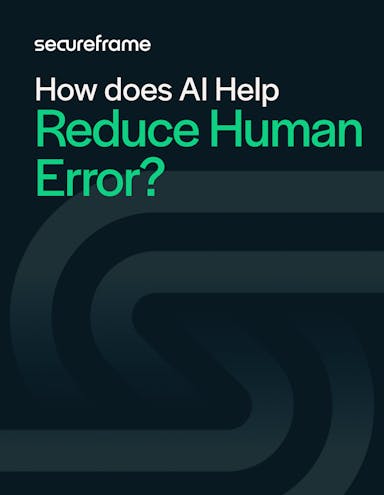
How Does AI Reduce Human Error in Cybersecurity and IT Compliance?
This ebook provides a high-level overview of the crucial role AI is playing in mitigating risks of data breaches, regulatory violations, financial losses, and more, using examples of real-world applications in cybersecurity and IT compliance.
How artificial intelligence is streamlining security and regulatory compliance
Similarly, AI and ML advances are being used to bolster security and compliance postures.
- Enhancing cybersecurity defenses: AI is pivotal in developing advanced cybersecurity measures. It can learn from ongoing cyber threats and adapt to new strategies employed by cybercriminals, continually strengthening an organization's defenses.
- Third-party compliance and regulatory checks: AI can ensure that third parties comply with relevant laws and regulations. It can monitor changes in regulatory frameworks and automatically assess the compliance status of vendors, reducing the risk of legal penalties.
- Automated security patching: AI can continuously monitor software and systems for vulnerabilities and automatically apply patches and updates to fix those vulnerabilities. AI systems can also prioritize patching based on the severity of the vulnerability and the criticality of the system affected, significantly reducing the window of opportunity for attackers.
- Stronger password security: AI can analyze password strength and automatically enforce an organization’s password policies, prompting users to change passwords at optimal intervals and suggesting strong, unique passwords.
- Incident response and threat containment: AI tools can quickly detect behavioral anomalies and security breaches and automate initial response actions, such as isolating affected systems, to contain the threat. After an incident, AI can analyze the attack to speed up resolution, aid in business continuity, and prevent similar attacks.
- Generative AI for policy creation: Tools like Comply AI can help teams quickly draft security policies by analyzing existing regulations, industry standards, and business needs and generating policy drafts that are both compliant and tailored to the organization. AI can also help keep policies updated by continuously monitoring for changes in compliance requirements and best practices.
- Machine learning for automated security questionnaire responses: Security questionnaires are a standard part of the vendor procurement process, allowing organizations to assess third-party security. But responding to questionnaires can be tedious and time-consuming, taking focus away from higher-priority tasks.
AI tools can streamline the process by ingesting completed questionnaires and analyzing that information to quickly generate responses. Secureframe’s Questionnaire Automation, for example, pulls data from the Secureframe platform and information from an internal Knowledge Base to automate responses to new questionnaires. SMEs can review and edit responses, then export the completed questionnaire in its original format to send back to prospects and customers. AI also ensures consistency and accuracy in questionnaire responses, saving time and reducing human error. - Tailored remediation guidance: AI applications are helping security teams remediate vulnerabilities by offering step-by-step remediation guidance. For example, Comply AI uses infrastructure as code to generate remediation guidance that’s tailored to the user’s cloud environment. Users can quickly and effectively fix failing controls to improve test pass rates and strengthen their security and compliance posture.
- Customized security awareness training
AI can analyze employee behavior to identify areas where security training is needed and develop targeted training programs. - Continuous compliance monitoring:
AI systems can monitor compliance with security policies and regulatory requirements in real-time, alerting compliance teams of nonconformities and failing controls so they can take action.
Recommended reading
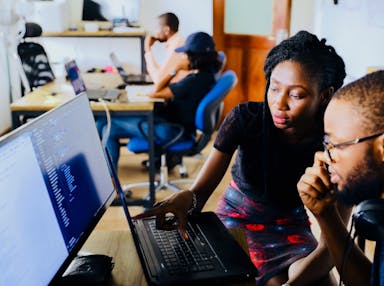
35+ AI Statistics to Better Understand Its Role in Cybersecurity [2023]
Steps organizations can take today to navigate and manage AI risk
Managing AI risks requires a proactive and comprehensive approach. By taking these steps, organizations can not only mitigate the potential downsides of AI but also harness its full potential responsibly and ethically.
Incorporate AI risk assessments into your risk management methodology
Invite stakeholders from compliance, IT, legal, and HR to weigh in on AI risk. Experts at McKinsey recommend assessing potential risks to:
- Privacy: Privacy laws around the world define how companies can and can’t use data. Violating those laws can result in significant violation penalties and reputational damage.
- Security: New AI models have complex, evolving vulnerabilities such as data poisoning, where ‘bad’ data is introduced into the training set and impacts the model’s output.
- Fairness: Organizations must take steps to avoid inadvertently encoding bias in AI models or introducing bias by feeding the model poor or incomplete data.
- Transparency: If organizations don’t understand how the AI model was developed or how the algorithms work to create the output, it creates a black box. For example, if a consumer requests how their personal data was used under GDPR, your organization must know which models the data was fed into.
- Third-party risk: Building or implementing an AI model often involves third parties in aspects like data collection or deployment, introducing third-party risk.
Once you’ve identified AI risks, you can create a risk treatment plan to prioritize and mitigate them.
Properly evaluate third-party AI tools
Organizations must also do their due diligence for data protection and privacy by properly evaluating third-party AI tools:
- Review the company's privacy policy and security posture
- Find out whether the information you share with the AI tool could be added to large language models (LLM) or surfaced in responses to other users’ prompts
- Ask potential vendors for an attestation letter verifying that the tool’s security has been assessed by a qualified third party
Define acceptable use in AI policies
A formal AI policy helps ensure that AI is used in a way that aligns with the organization's strategic goals and ethical standards. The AI policy should:
- Define acceptable use of AI tools
- Explain the organization’s approach to using AI in an ethical manner and the steps taken to combat bias and promote transparency
- Outline practices for data collection, management, storage, and use that satisfy any applicable data privacy regulations and compliance requirements
- Explain the processes put in place to monitor the effectiveness of AI tools and the AI policy itself
- Establish who will be responsible for enforcing the AI policy and keeping it up to date
Act now to prepare for future AI regulations
Organizations might be subject to multiple AI regulations based on industry, products and services, and customer base, making compliance complex. But the core focus of many AI regulations is similar: promoting transparency, accountability, privacy, and fairness when developing and deploying AI tools. Keeping those principles top of mind can help organizations and compliance teams stay ahead of the curve.
Security and compliance teams can prepare for future regulations now by:
- Implementing AI policies that clearly define acceptable use of AI
- Only allowing vetted and approved AI apps to be used on company devices
- Training personnel on the proper use of AI tools, including what data they can and cannot input into AI solutions and under what circumstances
- Gathering and maintaining documentation related to the use and development of AI within the organization, such as vendor contracts, risk assessments, and internal audit reports
Pair AI with GRC automation to reduce security and compliance risk
According to research by IBM, organizations with extensive use of both AI and automation experienced a data breach lifecycle that was over 40% shorter compared to organizations that have not deployed these technologies. That same research found that organizations with fully deployed security AI and automation save $3.05 million per data breach compared to those without — a 65.2% reduction in average breach cost.
Organizations that implement AI and security automation platforms together are in the strongest position to defend against emerging risks and a shifting regulatory compliance landscape.

Harness the power of AI for risk and compliance
The significance of AI in enhancing cybersecurity is only growing. By leveraging the power of AI and security automation, companies can strengthen and streamline their risk management and compliance processes to boost business resilience, operational efficiency, and security.
At Secureframe, we’ve incorporated cutting-edge developments in artificial intelligence and machine learning into our GRC automation platform, driving innovation and empowering security, risk, and compliance teams.
- Comply AI for Remediation provides AI-powered remediation guidance to help speed up cloud remediation and time-to-compliance.
- Comply AI for Risk automates the risk assessment process to save you time and reduce the costs of maintaining a strong risk management program.
- Comply AI for Policies leverages generative AI so you can save hours writing and refining policies.
- Secureframe’s questionnaire automation leverages AI so you can quickly answer security questionnaires and RFPs with more than 90% accuracy.
To learn more about how Secureframe uses automation and AI to improve security and compliance, schedule a demo with a product expert.
Use trust to accelerate growth
FAQs
How can AI be used in compliance?
- Automated Monitoring and Reporting: AI can continuously monitor compliance with regulations and internal policies, automatically generating reports and alerts in case of deviations.
- Regulatory Change Management: AI can track and analyze changes in regulatory requirements, ensuring that an organization's practices remain compliant.
- Data Analysis for Compliance Insights: By analyzing large volumes of data, AI can identify patterns and insights that support compliance efforts, such as detecting potential areas of non-compliance.
- Contract and Document Analysis: AI can review contracts and other legal documents for compliance with regulations and internal standards.
How can AI be used in risk management?
- Predictive Risk Analysis: AI can predict potential risks by analyzing historical data and identifying trends.
- Real-Time Risk Assessment: AI can assess risks in real time, providing immediate insights into emerging threats.
- Risk Data Management: AI can enhance the quality and efficiency of risk data collection, organization, and analysis.
- Scenario Modeling and Stress Testing: AI can simulate various risk scenarios, helping organizations prepare and mitigate potential impacts.
How do artificial intelligence and big data affect compliance and risk management?
- Enhanced Data Processing Capabilities: The combination of AI and big data allows for the processing of vast and complex datasets, providing deeper insights for risk and compliance management.
- Proactive Compliance and Risk Strategies: AI’s predictive capabilities enable organizations to adopt more proactive approaches in identifying and addressing compliance and risk challenges.
- Improved Accuracy and Efficiency: AI and big data can improve the accuracy and efficiency of compliance monitoring and risk assessment processes.
- Dynamic Adaptation: They enable dynamic adaptation to changing regulations and risk landscapes, keeping organizations agile and resilient.
Will compliance be replaced by AI?
AI is more likely to augment compliance functions rather than replace them. It enhances the ability to monitor, report, and respond to compliance issues but cannot fully replace the nuanced decision-making and strategic planning carried out by human experts. Compliance involves understanding complex legal and ethical considerations, which still require human judgment and oversight.
Why would AI need to be subjected to compliance regulations?
- Ethical and Legal Standards: To ensure AI operates within ethical and legal boundaries, especially regarding privacy, data protection, and non-discrimination.
- Trust and Transparency: Compliance regulations help maintain public trust and transparency in AI operations, which is vital for its acceptance and integration into society.
- Avoiding Harmful Outcomes: Regulations aim to prevent potential harmful outcomes of AI, such as biased decision-making, misuse of personal data, or unintended consequences that could arise from autonomous AI operations.
- Ensuring Accountability: Compliance ensures that there is accountability for AI decisions and actions, particularly in high-stakes areas like healthcare, finance, and law enforcement.