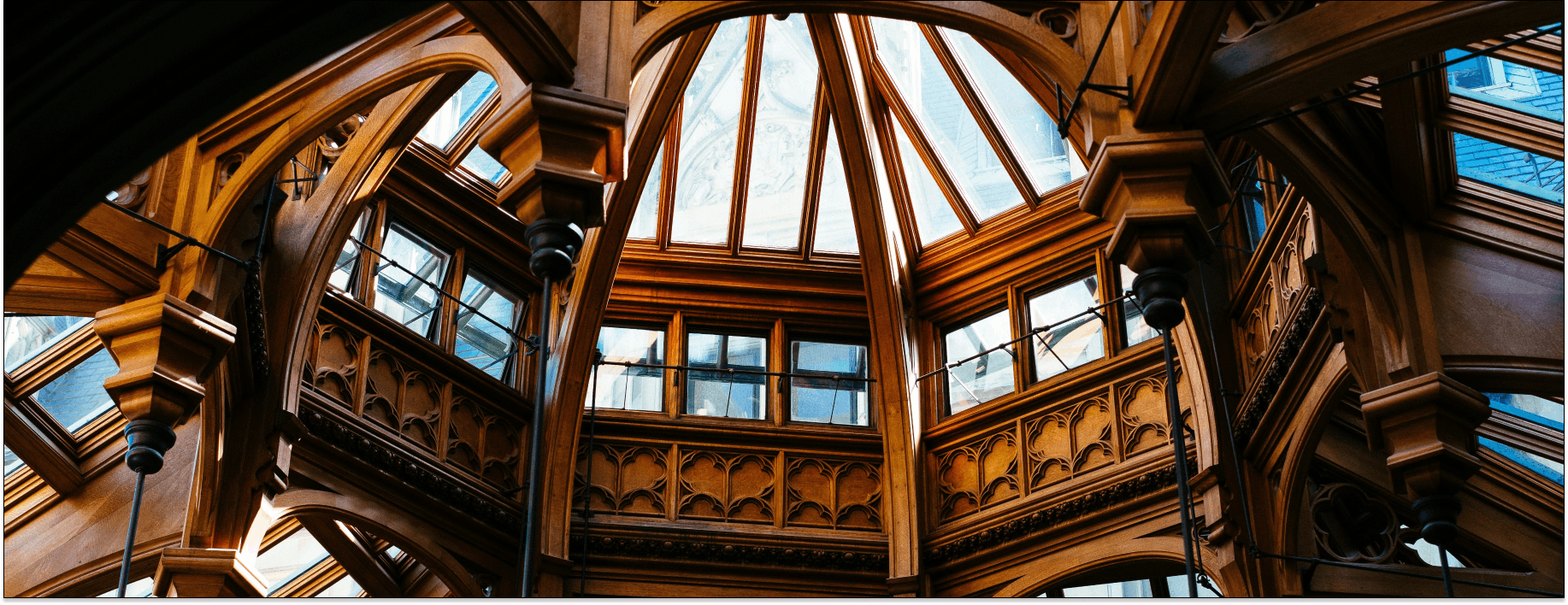
Comparing AI Frameworks: How to Decide If You Need One and Which One to Choose
72% of organizations are now regularly using AI technologies — yet 44% of organizations have experienced negative impacts from AI, including data privacy concerns and biases in AI models.
As the adoption of artificial intelligence tools skyrockets, security has emerged as a critical concern, from data breaches to ethical dilemmas. To address these challenges, a variety of AI frameworks have been developed, each designed to provide guidance and best practices for managing AI security risks.
But many businesses are left with pressing questions, such as: What is an AI framework exactly? What are the differences between them? Does my organization need to adopt one? If yes, which one? Where do we start?
Let’s dive into the essentials of the major AI frameworks to answer these questions and more.
What is an artificial intelligence cybersecurity framework, and do you need to adopt one?
As AI and machine learning continue to transform industries and reshape economies, organizations and government officials are recognizing the need for organized frameworks to manage the unique security risks associated with AI. The introduction of the EU AI Act, along with similar initiatives worldwide, underscores the urgency of regulating AI and establishing strong AI governance practices.
AI frameworks provide a structured approach to identifying, assessing, and mitigating the risks associated with AI, ensuring that organizations can leverage the technology’s potential while safeguarding against its pitfalls.
Let’s examine some benefits of adopting a formal AI framework:
1. Guidance for establishing strong AI governance
An AI framework offers comprehensive guidance for establishing robust AI governance and implementing security best practices. These frameworks serve as blueprints for organizations, helping them set up the necessary structures, policies, and procedures to manage AI effectively.
By following a well-defined framework, organizations can ensure that their AI systems are designed, developed, and deployed with security in mind, minimizing risks and enhancing the overall resilience of their AI initiatives.
2. Address unique risks associated with AI
AI introduces a new set of risks that are distinct from those typically encountered in organizations. These include algorithmic biases, adversarial attacks, and model vulnerabilities, among others.
An AI framework provides a structured approach to managing these unique risks, offering specific tools and strategies to identify and mitigate them. Without such a framework, organizations might struggle to navigate the complex landscape of AI risks, potentially leaving themselves exposed to unforeseen vulnerabilities.
3. Reduce the likelihood of data breaches introduced by AI
AI systems often rely on vast data sets, some of which may contain sensitive information or personally identifiable information. If not properly managed, these systems can become targets for cyberattacks, leading to significant data breaches.
By adopting an AI framework, organizations can implement robust data protection measures, such as encryption, access controls, and secure data handling practices, reducing the likelihood of breaches and ensuring that data is safeguarded throughout the AI lifecycle.
4. Ensure personnel understand the risks and opportunities of AI
One of the key benefits of an AI framework is that it helps ensure all personnel within an organization understand the risks and opportunities associated with AI. These frameworks often include training and awareness components that educate employees on how to develop, deploy, and use AI tools responsibly and securely. This not only enhances the security of AI systems but also empowers employees to leverage AI in a way that maximizes its benefits while minimizing risks.
5. Simplify compliance with emerging AI regulations
As AI continues to evolve, so too does the regulatory landscape surrounding it. The EU AI Act, along with other emerging laws and regulations, imposes new requirements on organizations using AI. Navigating this complex and ever-changing legal environment can be challenging.
An AI framework simplifies compliance by providing clear guidelines and best practices that align with regulatory requirements. This helps organizations stay ahead of legal obligations, avoid potential fines, and maintain their reputation in a rapidly evolving market.
To determine whether your organization would benefit from implementing an AI framework, you can ask yourself these questions:
- How extensively does our organization use AI tools? Are AI technologies integral to our operations, decision-making, or customer interactions? How critical are these AI systems to our business processes?
- What types of data do our AI systems handle? Do our AI models process sensitive, proprietary, or personally identifiable information (PII)? How do we currently secure the data used for training and deploying AI models?
- What are the potential risks associated with our AI systems or usage? Have we identified the specific risks AI systems could introduce, such as bias, adversarial attacks, or model vulnerabilities? How could these risks impact our organization, customers, or stakeholders if not properly managed?
- How well-prepared is our organization to manage AI-related security incidents? Do we have protocols in place for detecting, responding to, and mitigating AI-related security breaches or incidents? How quickly could we recover from an AI-related security incident, and what would the consequences be?
- Do we have the necessary expertise in AI within our organization? Does our security team have the knowledge and skills required to address the unique security challenges posed by AI? Are we equipped to continuously monitor and improve the security of our AI systems?
- Are we compliant with relevant AI regulations and standards? Are there emerging AI regulations or security standards, such as the EU AI Act, that our organization must comply with? How confident are we in our ability to meet these legal and regulatory requirements?
- How do we ensure ethical use of AI in our organization? Do we have a framework in place to ensure that our AI systems are fair, transparent, and aligned with ethical principles? How do we manage potential biases and ethical risks in our AI models?
- What is our level of AI governance and oversight? Do we have clear governance structures and accountability mechanisms for overseeing AI development and deployment? How do we ensure that AI projects are aligned with our organizational values and objectives?
- How do we currently address the transparency and explainability of our AI systems? Can we explain how our AI systems make decisions, and do we communicate this effectively to users and stakeholders? How transparent are our AI processes, and do we provide mechanisms for auditing and accountability?
- What is the potential impact of AI failures or data breaches on our organization? What could be the financial, reputational, or operational consequences if our AI systems were compromised? How important is it to our organization to mitigate these risks proactively?
If these questions reveal gaps in your current AI practices, consider implementing an AI framework to help you address these concerns systematically.
The next question to consider is which framework to adopt. Let’s examine some of the most prominent AI frameworks and the types of organizations they are most applicable to.
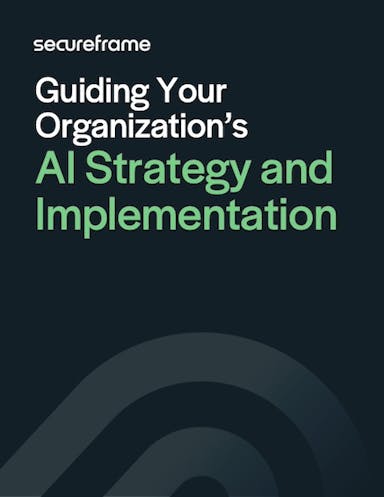
Guiding Your Organization's AI Strategy + Implementation
Learn best practices cybersecurity leaders can use to effectively implement AI while addressing concerns related to transparency, privacy, and security.
Which AI framework fits your needs? Comparing prominent AI frameworks
Since AI is a relatively new field, these AI frameworks still in their infancy/ It can be a significant challenge understanding the differences between them and their overlapping guidelines and standards. And because so many of the AI frameworks share similar goals, it can be difficult to discern which one is the best fit for your organization.
Below, we’ll share an overview of the major AI frameworks to understand their specific use cases and help you choose the framework—or combination of frameworks—that best aligns with your goals.
Framework | Governing body | Purpose | Applicable to | Certification? | General requirements |
---|---|---|---|---|---|
NIST AI RMF | National Institute of Standards and Technology (NIST) | Guide organizations in managing AI-related risks | Organizations of all sizes using AI | Voluntary | Focuses on risk management, transparency, accountability, and continuous improvement |
ISO 42001 | International Organization for Standardization (ISO) | Establish a comprehensive AI management system | Organizations implementing AI systems | Certification | Requires setting up an AI management system with policies, risk management, and continuous improvement processes |
OECD AI Principles | Organization for Economic Cooperation and Development (OECD) | Promote responsible stewardship of AI | Governments, organizations, and stakeholders using AI | Voluntary | Emphasizes human rights, fairness, transparency, and accountability in AI use |
IEEE AI Ethics Guidelines | Institute of Electrical and Electronics Engineers (IEEE) | Ensure AI systems align with ethical principles | Engineers, policymakers, and organizations developing AI | Voluntary | Promotes ethical AI design, focusing on human rights, transparency, and accountability |
British Standards Institution AI Standard | British Standards Institution (BSI) | Guide the ethical design and application of AI and robotics | Organizations designing or implementing AI and robotic systems | Voluntary | Ethical risk assessment and mitigation, human-centric design, and continuous improvement |
Google Secure AI Framework | Provide security best practices for AI | Organizations developing and deploying AI systems | Voluntary | Focuses on secure design, data protection, model integrity, and compliance | |
OWASP AI Security and Privacy Guide | Open Web Application Security Project (OWASP) | Offer best practices for securing AI and protecting privacy | Organizations designing or implementing AI and robotic systems | Voluntary | Threat modeling, data security, model protection, and incident response |
ISO 23894 | International Organization for Standardization (ISO) | Providee guidelines for ethical AI governance | Organizations involved in AI governance | Certification | Governance structures, risk management, and ethical considerations |
IBM Framework for Securing Generative AI | IBM | Secure generative AI models and systems | Organizations using generative AI technologies | Voluntary | Model security, data protection, adversarial threat mitigation, and transparency |
NIST AI Risk Management Framework (AI RMF)
The NIST AI RMF is designed to help organizations manage the risks associated with the development, deployment, and use of AI systems. Released in January 2023, the framework emphasizes the development of trustworthy AI by addressing aspects like fairness, transparency, security, and privacy.
The core of the framework describes four functions designed to help organizations address the risks of AI systems: govern, map, measure, and manage.

The NIST AI RMF is a voluntary framework intended for a broad range of organizations. This flexibility allows organizations to tailor the framework to their specific needs, regulatory environments, and risk tolerances. By adopting the AI RMF, organizations can better navigate the complexities of AI technologies, ensuring they harness AI's benefits while mitigating potential harms.
ISO 42001
ISO 42001 provides a comprehensive framework for establishing, implementing, maintaining, and continuously enhancing an Artificial Intelligence Management System (AIMS), which consists of all the policies, procedures, and controls an organization implements to address AI risks. The international standard was created through the collaboration of global stakeholders, including technology companies, policymakers, and community organizations, and offers a way for organizations to showcase their commitment to excellence in AI governance.
Compliance with ISO 42001 involves implementing policies and procedures for developing and deploying trustworthy AI, following the Plan-Do-Check-Act methodology. Rather than focusing on specific AI applications, it provides a practical framework for managing AI-related risks and opportunities across an entire organization.
Unlike NIST AI RMF, ISO 42001 is a certifiable AI framework. Organizations can demonstrate compliance by completing a third-party audit with an accredited certification body.
Similar to ISO 27001, the certification process for ISO 42001 involves a Stage 1 audit (documentation review), Stage 2 audit (assessment of AIMS implementation and effectiveness), certification decision, annual surveillance audits, and recertification audits every three years.
Recommended reading
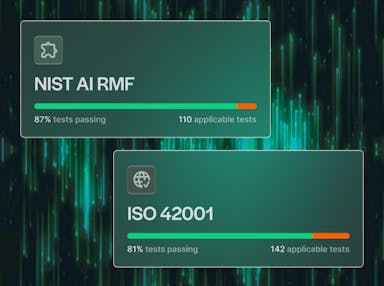
Secureframe Introduces Support for NIST AI RMF and ISO 42001
OECD AI Principles
Developed by the Organization for Economic Cooperation and Development (OECD) to promote the responsible development and use of AI, these principles were adopted in 2019 and have been endorsed by over 40 countries, making them a significant international framework for AI governance.
The AI principles are:
- Inclusive growth, sustainable development, and well-being: AI should contribute to economic growth, social well-being, and environmental sustainability. It should be used in ways that benefit society as a whole, including marginalized and vulnerable groups.
- Human-centered values and fairness: AI systems should be designed and used in ways that respect human rights, dignity, and autonomy. This includes ensuring fairness, preventing bias, and avoiding discrimination in AI outcomes.
- Transparency and explainability: AI systems should be transparent and understandable to users and stakeholders. This includes providing clear information about how AI decisions are made and ensuring that AI systems can be audited and scrutinized.
- Robustness, security, and safety: AI systems should be robust, secure, and safe throughout their lifecycle. This involves rigorous testing, monitoring, and risk management to prevent harm, including unintended or malicious use.
- Accountability: Organizations and individuals responsible for AI systems should be held accountable for their proper functioning and impact. This includes establishing clear lines of responsibility and ensuring that there are mechanisms in place to address issues that arise from AI use.
In addition to the core principles, the OECD also provides guidelines for governments and organizations on how to implement these principles effectively. For example, an organization developing AI-driven healthcare solutions might ensure that its products are accessible to diverse populations, including underserved communities. They would also implement processes to regularly assess the accessibility and impact of their AI products and algorithms on different demographic groups to ensure that the benefits of AI are equitably distributed.
IEEE AI Ethics Guidelines
These guidelines are part of the broader initiative by the IEEE Global Initiative on Ethics of Autonomous and Intelligent Systems, which seeks to prioritize human well-being in the creation of AI and autonomous systems.
The primary goal of the IEEE AI Ethics Guidelines is to ensure that AI technologies are developed and deployed in ways that are ethical, transparent, fair, and beneficial to society. For example, that AI systems are designed to respect fundamental human rights such as the right to privacy and freedom of expression. By adhering to these guidelines, organizations can build trust in their AI systems, mitigate risks, and promote responsible AI across different sectors.
The IEEE guidelines are widely respected and serve as a reference point for policymakers, engineers, and organizations looking to navigate the ethical challenges posed by AI and autonomous systems.
British Standards Institution AI Standard
The BSI AI Standard provides a structured framework for identifying, assessing, and mitigating ethical risks in the design and use of AI and robotic systems. This includes potential impacts on privacy, safety, human rights, and societal well-being. It also categorizes ethical hazards into different types, such as physical, societal, and environmental risks. This helps organizations assess how AI and robotic systems might pose ethical challenges, such as violating privacy, causing harm, or creating social inequality.
Organizations are encouraged to integrate the framework into their AI development processes, particularly during the design and testing phases. This involves conducting ethical risk assessments, applying the standard's guidelines to mitigate identified risks, and continuously monitoring the ethical performance of AI systems.
Google Secure AI Framework (SAIF)
This is a security framework developed by Google to guide the development and deployment of secure AI systems, from data collection and model training to deployment and ongoing monitoring. By implementing the principles outlined in SAIF, such as security by design, data privacy, and secure deployment and monitoring, organizations can build AI systems that are resilient to attacks and aligned with security and privacy standards.
OWASP AI Security and Privacy Guide
An initiative by the Open Web Application Security Project (OWASP), the AI Security and Privacy Guide provides best practices and recommendations for securing AI systems and ensuring they respect privacy. OWASP is well-known for its focus on web security, and this guide extends its mission to the realm of AI, addressing the unique challenges and risks associated with AI technologies.
The guide offers a comprehensive set of best practices for securing AI systems and protecting privacy, such as threat modeling, data security, adversarial defenses, model integrity, privacy-preserving techniques, ethical considerations, and incident response.
ISO/IEC 23894
While still under development, ISO 23894 will focus on AI system governance, aiming to provide comprehensive guidelines and best practices for organizations to ensure that their AI technologies are managed responsibly and ethically. This includes defining roles and responsibilities, setting policies, and ensuring oversight of AI development and deployment.
A significant focus of ISO 23894 is on managing the risks associated with AI systems. This includes identifying potential ethical, legal, operational, and regulatory compliance risks and providing strategies to mitigate them throughout the AI lifecycle.
While ISO 42001 provides a holistic management system framework for AI, ISO 23894 focuses more narrowly on the governance and ethical aspects of AI systems. Both standards are complementary, with 42001 offering a broader management perspective and 23894 diving deeper into governance and ethics.
IBM Framework for Securing Generative AI
This a comprehensive set of guidelines and best practices designed by IBM to help organizations address the unique security challenges of generative AI models, such as those used in natural language processing, image generation, and other creative applications.
The framework emphasizes the importance of securing the data used to train gen AI models, as this data often contains sensitive or proprietary information. Organizations are encouraged to use data anonymization, encryption, and secure data handling practices to protect training data and ensure compliance with privacy regulations.
Generative AI models can be vulnerable to adversarial attacks, where inputs are designed to manipulate the model into producing harmful or misleading outputs. In response, IBM’s framework also advises organizations to implement detection mechanisms, use adversarial defense techniques, and continuously test models against potential threats to mitigate the risks of adversarial attacks. The framework also suggests incorporating fairness checks, bias detection tools, and ethical guidelines into the development process to ensure that generative AI models produce content that aligns with societal values and ethical standards.
How to choose the right secure AI framework for your business
Choosing the right AI framework for your organization can be a daunting task. These questions are designed to guide you through the process, helping you identify the framework that aligns most closely with your organization’s specific goals, risk profile, and regulatory environment.
By answering a series of targeted questions, you’ll be able to navigate the complexities of AI security and make an informed choice that supports your AI initiatives while safeguarding against potential risks.

Ultimately, if you need risk management and comprehensive governance, choose NIST AI RMF or ISO 42001. For a strong focus on ethical AI and human-centric design, IEEE AI Ethics Guidelines or ISO 23894 might be ideal. If security and privacy are your primary concerns, consider Google Secure AI Framework, OWASP AI Security and Privacy Guide, or the IBM Framework for Securing Generative AI. If you require a formal certification, ISO 42001 or ISO 23894 are likely the best fit. And if aligning with global ethical standards is key, OECD AI Principles or British Standards Institution AI Standard are good options.
Achieve AI framework compliance fast with automation
No matter which AI framework — or frameworks — you decide to adopt, using a compliance automation tool like Secureframe can make the process significantly faster, easier, and more efficient.
- Out-of-the-box support for NIST AI RMF and ISO 42001, plus the ability to create custom frameworks to fit any AI framework.
- Continuously monitor your tech stack to identify vulnerabilities and remediate failing security controls in real-time
- Automatically collect evidence to cut hundreds of hours of manual work for internal or external audits
- Use AI-powered risk assessment workflows to produce inherent risk scores, treatment plans, and residual risk scores
- Simplify multi-framework compliance with ComplyAI for Control Mapping, which intelligently suggests control mappings across applicable frameworks
To see how Secureframe's AI innovations can streamline compliance for your organization, reach out to schedule a demo with one of our product experts.
Use trust to accelerate growth
FAQs
What is the NIST AI framework?
The NIST AI Framework, formally known as the NIST AI Risk Management Framework (AI RMF), is a set of guidelines developed by the National Institute of Standards and Technology (NIST) to help organizations manage the risks associated with artificial intelligence (AI) systems. The framework provides a structured approach for organizations to assess, mitigate, and monitor risks related to AI, focusing on aspects such as transparency, accountability, fairness, and security.
What are AI frameworks?
AI frameworks are structured sets of guidelines, best practices, and principles designed to guide the development, deployment, and governance of artificial intelligence systems. These frameworks help organizations manage the unique risks associated with AI, such as bias, security vulnerabilities, and ethical concerns, while also ensuring compliance with legal and regulatory requirements.
How can AI be used for cybersecurity?
AI can be leveraged in cybersecurity to enhance the detection, prevention, and response to cyber threats. AI-powered tools can analyze vast amounts of data to identify patterns and anomalies that may indicate a security breach or malicious activity, as well as automate routine cybersecurity tasks, such as monitoring network traffic, identifying vulnerabilities, and responding to incidents.